How AI Will Evolve In Healthcare
By Shailesh Tiwari, MST Solutions
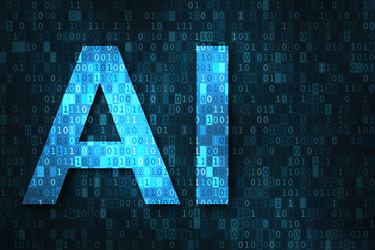
Artificial intelligence (AI) stands to become one of the most transformational technologies of our time. Its practical application throughout various industries—particularly healthcare—is growing. From chronic to acute diseases to clinical trials and drug development, the opportunities for a more precise and efficient intervention driven by AI are endless.
While it may take some time to realize, the growing impact of AI cannot be dismissed—77 percent of healthcare executives report their organizations have already accelerated investments in AI and Big Data, according to a survey by NewVantage Partners. What does this mean to the future of healthcare? Should healthcare IT professionals consider AI a vital part of the roadmap? If so, what are the challenges and opportunities that come with it, specifically in relation to the healthcare industry?
What Is AI And How Is It Being Used?
AI, at its highest level, describes a machine’s ability to mimic the cognitive function of the human mind, such as learning and problem solving. It is the use of technology to help machines sense, act and learn (machine learning).
In healthcare, AI algorithms and software are used to analyze complex medical data, and to arrive at conclusions without human input. Currently, AI is supporting in areas such as the detection and diagnosis of diseases at early stages, medical image processing, precision medicine, health monitoring, treatment design, virtual assistance, robot-assisted surgeries, drug creation and cost reduction, amongst numerous other applications.
In fact, Healthcare IT News conducted a survey at the end of 2018 in which they asked health IT, informatics, business and clinical professionals where AI is delivering value. Sixty three percent of respondents said AI is delivering value in specialty care in areas such as radiology, pharma, pathology, etc., and just over 60 percent said it’s delivering value in patient engagement by way of telehealth and remote health monitoring. As well, 77 percent either use or plan to use AI to support clinical decision making, and 66 percent use it to glean insights from Big Data sets.
Challenges And Limitations Of AI In Healthcare
Still, even with all of its promising applications, there are concerns with AI as it applies to the medical industry.
Privacy and ethics. First, and foremost, is the concern about patient privacy and ethics. A question on the minds of most is who owns the patient data needed for the AI technology? While HIPPA protects patient health data when it comes from organizations that provide healthcare services, the law does not carry over to the tech companies building and using AI technologies.
For example, since companies such as 23andMe and Ancenstry.com recently came under fire for selling customer DNA-related data to pharmaceutical companies and universities for research. However, since they are not classified as healthcare providers or entities, they are not required to follow HIPPA laws. On the other side of the coin, ethical and legal questions are raised when thinking about whether hospitals should be allowed to provide patient data to AI companies—how can a patient’s right to privacy be protected?
Fragmented. For AI to work effectively, it relies on high-quality data sources. Yet this remains a tremendous barrier in healthcare today with data being siloed in legacy systems that don’t talk to one another. Plus, the privacy concerns about patient data sharing can hinder obtaining large data sets. So while large amounts of data may be available via electronic health records (EHRs) and health monitoring devices, it needs to be structured, coded and de-identified properly for AI to utilize the information.
Quality data. Another concern is that predictive analysis is just that—a prediction based on inputted data. But the power of predictive analysis is directly correlated to the quality of data feeding it. Because data remains highly fragmented and often is in multiple formats, much of the data is incomplete and inconsistent. Because of this, there has been much concern over AI’s potential to draw the wrong conclusions, particularly since it doesn’t have the capability to weigh the costs and consequences of false positives or negatives the way a physician might. On top of it, electronic health records and billing claims are often written with broad categories and can be difficult to interpret. Not to mention many entries include errors or omissions, whether inadvertent on behalf of the doctor, or whether a patient did not share crucial information.
Data labeling. For deep learning to occur, thousands of data records and labeled examples using consistent terms are required for models to perform classification tasks. Right now, most of that information, is recorded using inconsistent terms or is very broad. Still, labeling and training the data remains a very manual task and also can open the door to bias and errors in the learning algorithm.
With so many potential challenges and current limitations, where does that leave AI in the healthcare equation?
What Is The Future Of AI In Healthcare?
Despite current perceived and actual barriers, AI is dramatically changing the way healthcare is delivered and it has already shown to have a positive impact on certain healthcare outcomes. For these reasons, we can count on seeing AI being used more frequently in the following areas:
- Predictive maintenance. As more pressure continues to be put on healthcare providers and leaders to achieve greater profitability, implementing predictive maintenance models for proactively repairing and servicing medical technology and devices can reduce unplanned system and operational downtime by anticipating potential failures before they become an issue.
- Data security. As security threats continue to rise, AI in tandem with predictive analytics, will become important tools in monitoring data access patterns and calculating real-time risk scores to deny or grant access.
- Automation. Some EHR platforms are utilizing AI to bring intuition and automation to rote processes that take up a provider’s valuable time. This also can improve the provider experience.
- Proactive intervention. Aggregating information across multiple devices and strategically using that data, particularly as consumer smart devices increasingly enter the healthcare fold, has traditionally been a challenge. With AI, it can enhance the ability to identify potential anomalies, health risks or potential complications and plan intervention before it becomes an issue.
- Expanding access to care. Delivering care to remote areas and developing regions remains a primary challenge within healthcare. However, through the use of AI-diagnostic and imaging tools, patients in remote areas can undergo scans for specific diseases, for instance, without the need for a specialist or provider being on-site.
AI has already been used to support clinical decision making by offering predictive outcomes based on patterns and trends, as well as in care coordination and planning. This will only continue to expand in healthcare as more data is collected.
How to Prepare for AI
As AI becomes a more prominent part of the medical industry, healthcare organizations will need to strategically plan how to organize and streamline their technology, processes and people to integrate the newest advancements. Identifying specific use cases is a good place to start to determine if data already exists and the maturity of that data.
Introducing AI technology also comes with an investment resources –– time, budget and team knowledge. You’ll want to be sure you have the right people in place –– data scientists, system architects, and even internal champions, among others –– who will help with the implementation, change management and maintenance.
As you evaluate the right technology for your organization, you’ll also want to consider whether the solution can scale with your business, how data is trained and what data is used in the training, how data bias and “dirty” data is prevented, and how it integrates feedback into its model. These are important for avoiding some of the aforementioned limitations and challenges of AI.
Ultimately, there is a lot to consider before introducing AI to your organization. Be sure to evaluate the impact to all stakeholders ––patients, providers, business leaders, etc. Will the endeavor pay off? And how long will it take to realize that payoff?
Shailesh is a Technical Architect at MST Solutions who has experience developing and delivering innovative solutions for healthcare companies and levering AI and IoT to build patient-centric solutions and improve business outcomes.