HIT, AI, And Machine Learning: A Reality Check
By Russell Olsen, WebPT
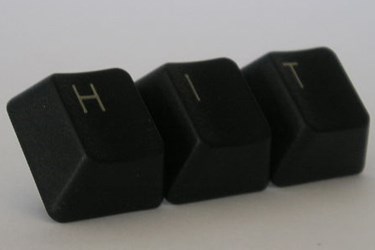
In the healthcare IT space, there are two buzzwords du jour: artificial intelligence (AI) and machine learning (ML). These concepts—which are often used interchangeably, but have distinct meanings—have been linked to everything from drastically changing future patient experiences (undoubtedly true) to completely replacing physicians with robots (not likely).
As it stands, many healthcare companies are making claims that their products integrate AI and ML, but that doesn’t necessarily mean they’re doing it well. So, which claims are science—and which ones are science fiction? With the AI healthcare market estimated to hit $6.6 billion by 2021—and one in five U.S. consumers saying that they’ve already received healthcare services that leverage “artificial intelligence”—it’s time for us, as leaders, to augment our knowledge about AI and ML in healthcare.
Read on to learn the nuances of AI and ML—as well as the difficulties many organizations face when attempting to incorporate them into their offerings and the key questions to ask when vetting vendors that tout these technologies as a selling point for their products.
The Difference Between Machine Learning And Artificial Intelligence
Increasingly driven by regulation, automation, and—let’s face it—frustration, the healthcare arena is a constantly shifting world for providers and patients alike. So, it’s not surprising that the terms “artificial intelligence” and “machine learning” are erroneously being bandied about as synonyms. In truth, machine learning is an application of AI. Here’s the layman’s lowdown:
Artificial intelligence is an expansive concept that encompasses machines using algorithms (i.e., sets of rules for crunching data to solve problems) to carry out tasks in an “intelligent” way. Machine learning, a subset of AI, involves the capacity of a machine to take a data set, learn it, and then adapt the algorithm as it processes more data. In short, AI is incorporating human intelligence to power machines. ML is empowering computer systems to make decisions based on that intelligence.
If you take one thing away from the explanation above, let it be this: data is at the heart of the matter when it comes to successfully leveraging AI (and therefore, ML) in healthcare products. As nutritionists like to say, “garbage in, garbage out.” The same goes for AI. If the data a product uses is flawed, the resulting insights will be unreliable as well.
The Limitations Of Machine Learning
Headlines abound about the capacity for machine learning to transform the future of healthcare, and I’m certainly not here to dispel the powerful contributions it will make to our field. I do want to point out, however, that like every science, machine learning has limitations. And it’s crucial to consider these constraints when determining the feasibility of associated healthcare applications. They include:
- Quality data is essential. See my “garbage in, garbage out” comment above. The bottom line is that machines can’t make good decisions without good (i.e., clean) information.
- Large data sets are required. Machines learn to solve problems by training on data patterns or models. A data set that is too small will hamper the resolution of future complex problems.
- Operator error is not uncommon. When a machine learning application fails, the buck rarely stops with the algorithm. It’s more likely that a human has introduced an error into the training data, causing bias or some other systemic issue.
- Biases create self-fulfilling prophecies. Once an error or bias has been introduced into a machine learning environment, the system can continue to create new data that reinforces those biases.
- Healthy feedback loops are critical. Machine learning will always entail the integration of new data that wasn’t part of the original training set. Feedback loops—whereby a system’s output is vetted to eventually influence its input—play a primary role in continually ensuring accurate results. But if the feedback loop is poorly maintained―or affected by human error―you again have garbage going in and (you guessed it) garbage coming out.
An Example
Here’s a real-life example of some of the challenges associated with using ML in healthcare: imagine, for a moment, that your practice wanted to leverage ML to help determine patients’ optimal treatment plans. Your first challenge? You’d need a massive set of training data to create a model—specifically, one that doesn’t contain any inherent biases. Next, you’d need a completely different data set to validate the accuracy of your model. Finally, assuming you’ve made it this far, you would have to seek user (i.e., provider) feedback. Do providers agree with the treatment recommendations? What do you do if some providers accept the ML advice and others ignore it?
The takeaway: When a vendor claims its product is enhanced by machine learning, dig into the details—what does that actually mean? —and know that the process of integrating ML into your practice is going to be a long, winding road.
Due Diligence: Key Questions To Ask Technology Vendors
With the above limitations in mind, here are several questions to ask when selecting technology with so-called “AI” or “ML” functionality (keep in mind that these are just a starting point):
- Data: How is the model trained? Which data sets were used to train the model? What size are the data sets?
- Error rate: How does the vendor prevent dataset bias or feedback loop problems introduced by human error, data selection issues, or dirty data? What is the false-positive to detection rate ratio?
- Feedback process: What is the feedback loop for the model, and how is the model adjusted to reflect feedback it receives? In turn, how frequently does the model need updating? Does this influence accuracy in between updates?
- Scalability: In what ways does the machine learning solution scale as your business changes?
A Reality Check On Artificial Intelligence And Machine Learning In Healthcare
If it sounds like I’m negating the benefits of AI and ML-supplemented healthcare solutions, let me assure you that’s not the case. The U.S. healthcare system currently churns out an estimated one trillion gigabytes of data annually, and there are plenty of examples of how this type of technology can help providers care for their patients. But the truth is, machine learning lends itself more successfully to certain healthcare arenas—usually those that are standardized and have large amounts of data. For example, it has revolutionized the automation of administrative tasks, which could result in $18 billion in savings. Cardiology, pathology, and radiology are also good candidates for machine learning due to their extensive datasets and need for timely image analysis.
However, there also have been setbacks that suggest the technology has been a victim of hype. For example, one well-known ML system developer recently faced criticism for overoptimistic claims about where the solution would be by now. The problem? The same one facing providers of ML-based healthcare applications everywhere: it needed a large and specific data set to be trained. Unfortunately, that data is often hard to find or access—and it takes teams of experts to dissect and organize.
According to Thomas Fuchs—a computational pathologist at Memorial Sloan-Kettering Cancer Center in New York— “If you’re teaching a self-driving car, anyone can label a tree or a sign so the system can learn to recognize it. But in a specialized domain in medicine, you might need experts trained for decades to properly label the information you feed to the computer.” So, while we should all have our eyes on the future gains AI will deliver to healthcare outcomes, a respectful scrutiny of product claims and timelines from machine learning vendors is healthy.
When considering related applications for their own practices, health IT pros should arm themselves with a solid understanding of technological subtleties, realistic expectations about solutions’ potential constraints for our industry, and a list of targeted questions that will help weed out implementation roadblocks down the line. Weighing the pros and cons of AI, Eliezer Yudkowsky—cofounder of the Machine Intelligence Research Institute—might have summed it up best: “By far, the greatest danger of Artificial Intelligence is that people conclude too early they understand it.”
About The Author
Russell Olsen is VP of Innovation and Product Management at WebPT where he leads category design, product management, user experience, and product discovery—as well as applying disruptive innovation approaches to accelerate growth while solving customer and market problems. Russell brings with him deep experience in healthcare and growth companies and has delivered innovations impacting millions of lives over the course of his 15-year career at companies including Phytel and IBM Watson Health.