What Is The Value Of Converting EHRs Into Phenotypes Focused On Diseases?

By Christine Kern, contributing writer
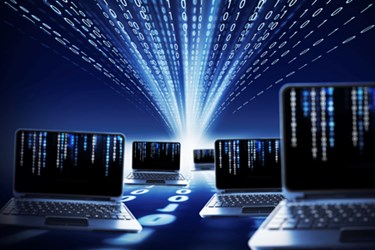
Researchers are looking to transpose diverse and massive health data into useful clinical tools.
By Christine Kern, contributing writer
A group of researchers at Georgia Tech have launched a four-year, $2.1 million NSF research project employing data analytics to develop algorithms and methods to convert EHR data into meaningful phenotypes focused on diseases and specific health traits. The goal is to mine useful data from the EHR landscape.
Initial EHR data and phenotype validation is being provided by Vanderbilt University, and resulting phenotypes will be refined and adapted in conjunction with data from Northwestern University to allow the information and data to be utilized across multiple health institutions.
A Georgia Tech press release explains that, while EHR “databases promise to serve as rich resources for clinical research, the data tends to be difficult, time-extensive, and costly to analyze.” That was the prompt for this new project funded by the National Science Foundation (NSF).
“As available now, databases of electronic health records are diverse and massive, but they are also messy and heterogeneous. There’s a lot of noise,” Jimeng Sun, associate professor at Georgia Tech’s School of Computational Science and Engineering, said in the release. “Our charge is to find ways to make the information more robust and easier to read, thus leading to meaningful clinical concepts without extensive labor and time.”
Sun leads the research team, which also includes Bradley Malin and Joshua Denny, associate professors of biomedical informatics and computer science at Vanderbilt; Joydeep Ghosh, professor of electrical and computer engineering at Texas; and Abel Kho, associate professor of medicine-biomedical informatics at Northwestern University.
Previously, attempts at creating phenotypes have been costly and time-intensive, making them inefficient. Denny explained, “Traditionally it takes six to 18 months to develop an algorithm for a single phenotype, which is too long. There is also a tremendous need for developing high-throughput phenotyping methods that can directly model the interactions among heterogeneous information sources.”
The current project includes a system to accurately and effectively identify patients with multiple symptoms and health traits for clinical research and developing predictive models for health studies.
The project can also provide effective phenotypes for genomic-wide association studies (GWAS). Currently, processes only allow health researchers to work with a single phenotype at a time, but this project is designed to create a process that will enable researches to quickly study multiple phenotypes simultaneously. The phenotypes can then also help analyze related specific risk information, such as key health factors exhibited by Type 2 diabetes patients.
The professors will also work to develop new health analytics curricula offered as a massive open online course (MOOC) and tutorial sessions at conferences.
The project abstract concludes that, “Overall, the proposed framework is expected to have a major impact on translational clinical research including clinical trial design, predictive modeling, epidemiology studies and clinical decision support.”