Using De-identification To Break The 80/20 Rule Of Health Data
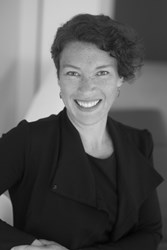
By Pamela Neely Buffone, Vice President of Product Management, Privacy Analytics
Recent initiatives supporting advanced medical research and precision medicine have underscored the importance of enabling access to health data to develop new ways to diagnose, treat, and potentially cure what ails us.
This demand for access to health data is driven by researchers and patient advocacy groups looking for better and faster ways to treat diseases and conditions. It can also come from regulatory entities that want better reporting and transparency concerning the results of clinical trials and medical device testing.
Pharmaceutical companies that receive federal funding to conduct clinical trial studies are often required to share results and have the data available to other researchers. Secondary analysis of clinical trial data can provide new insights into other therapies and provide a wider disclosure of adverse events.
One of the challenges to this growing demand for health data is that, while health organizations are collecting a rapidly increasing volume of data every day, they may only be capable of analyzing and sharing a small portion of that information. According to organizations such as Merrill Lynch and IBM, 80 percent of digital information, including health data, is unstructured.
Unstructured health data comes from many sources such as notes taken in a patient consultation or during a clinical trial, voice to text transcriptions, scanned documents, surveys and questionnaires, and email exchanges. While much of this information may be stored in EHRs, it is often free-form text rather than organized data in pre-defined fields. Think Word document versus Excel. Or junk drawer versus filing cabinet.
Trying to access, aggregate, and share this information has presented serious privacy concerns for health organizations. They must be able to identify and extract only the information from unstructured data that is valuable for research, analysis, and regulatory compliance while de-identifying the information that could expose individual patients, putting their privacy at risk.
While technologies exist to search unstructured data, the challenge many organizations face is finding a scalable method to achieve regulatory compliance and prevent unintended disclosure of any protected health information (PHI) that may be present in these massive data files. Manual methods for protecting privacy in unstructured data, such as searching for keywords and redacting information, are simply not feasible when dealing with millions of health records. As a result, many organizations are faced with the 80/20 rule of health data. With 80 percent of their data in unstructured files, they are left with the ability to access and share only the remaining 20 percent of their data contained in structured formats. This is a significant impediment to data sharing needed for medical innovation and discovery.
Combining NLP (natural language processing) functionality with de-identification methods and technologies that are compliant with regulations and globally accepted standards and guidelines can help solve this problem. Current standards on risk-based de-identification methodologies from HIPAA, HITRUST, the Institute of Medicine (IOM), PhUSE, the Council of Canadian Academies, as well as the EU Data Protection Directive 95/46/E. are already established to protect privacy. The next step is for organizations to adopt these standards and use technologies that scale the de-identification process, allowing them to effectively harvest valuable information from vast stores of unstructured data and share only what’s needed.
Organizations such as the National Institute of Health (NIH) and the Ontario Brain Institute (OBI) have already begun to do this. NIH currently de-identifies thousands of unstructured data records every day to allow researchers access to critical health information. OBI also provides scientists and clinicians with de-identified patient information from both structured and unstructured data sources to advance critical brain research.
The ability to de-identify PHI contained in unstructured files can allow organizations to unlock the other 80 percent of their health data assets that have been largely inaccessible for important secondary uses. It can provide access to exponentially more valuable information that can be combined with structured data to provide greater context, insight and knowledge. Unstructured health data must be de-identified, aggregated and shared in a responsible and compliant manner if we expect to achieve greater advances in treatments and cures sought by a wide range of healthcare stakeholders and several prominent and promising national initiatives.
About The Author
Pamela Neely Buffone is Vice President of Product Management for Privacy Analytics, where her mandate is to make the risk-based approach to de-identification more accessible to meet the dual needs of data utility and privacy protection. The focus of her career has been to make analytics more consumable and easy to use, thereby bringing the value of insight and discovery to more people across industries and domains. She can be reached at PBuffone@privacy-analytics.com.