Unravelling The Mysteries Of Medical Billing With Artificial Intelligence
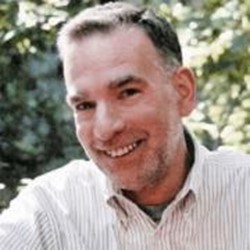
By David Bayer, Vice President, Compreno Group at ABBYY
Codes, Codes And More Codes
When you go to the grocery store and make a purchase, the receipt you receive at the end of your visit is fairly self-explanatory. Two dozen eggs, a carton of milk, three pounds of bananas — each with an itemized cost and totaled up at the bottom. It’s relatively easy to spot an error, such as seeing two cartons of milk on your bill when there is only one carton in your bag.
Compare this to a medical bill you might receive from a doctor or a hospital. A quick glance reveals a document of byzantine complexity resplendent with “test codes” and “CPT codes.” Spotting an error is very different.
Only One In 10 Medical Bills Is Accurate
The complexity of billing code knowledge required to understand a standard medical bill makes it no surprise a recent study found up to 90 percent of hospital bills contain errors totaling some $750 billion annually. This represents a huge challenge for healthcare insurance companies which need to ensure that medical providers are billing them correctly. Billing errors can originate from a variety of sources including:
- Upcoding – When a medical billing code is improperly changed to one representing a more severe diagnosis or treatment, e.g., coding for a name brand medication when a generic one was used.
- Unbundling – When charges that normally fall together under one billing code are listed separately, e.g., if multiple medical tests that should fall under that same medical code are billed separately.
- Duplicate Charges – When procedures or services are billed multiple times, e.g., if a doctor and a nurse indicate a particular medication was given without knowing the other had already billed for the service.
Many billing errors require detailed investigations. The vast majority of states have “prompt pay” regulations that require payment within a specified time period, sometimes in as little as 10 days.
Recovering the funds associated with this overcharging requires requesting the relevant patient medical records and having them reviewed by a clinician. The clinician correlates the patient’s treatment as detailed in medical records with the charges from the healthcare provider. This is a lengthy and expensive process, given clinicians are expensive resources and reviewing medical records is time consuming because they are usually provided in an unstructured textual form such as a Word document or PDF.
This service is typically performed by specialist third parties, called recovery audit contractors or RACs. These RACs are paid via a fee, usually a percentage of the funds recovered. The effectiveness of the RACs is heavily reliant on the skill of the clinician responsible for reviewing of medical records.
New Technology Can Ease The Pain
Today’s advanced text analytics technology can intelligently analyze the unstructured textual data contained in medical records, which can be used by clinicians to accurately extract entities, events, and facts. Powered by cutting-edge natural language processing technology, certain artificial intelligence tools on the market are able to recognize specific words while deciphering their meaning and relationship to one another. These types of technologies hold great promises of efficiency for health IT departments. In fact, Frost & Sullivan predicts healthcare provider and consumer spending on AI tools will reach more than $6 billion by 2021.
AI technologies in healthcare provide the ability to create custom user dictionaries and ontologies, tailored to extract the complex entity relationships that exist in medical records and their corresponding bills. These tools can also be tailored to the particular specialization of recovery audit contractors including:
- CPT Codes
- ICD Codes
- Drugs
- Dosages
- Medical Tests
This provides the clinician with a timeline of events contained in the medical records and tells the story of the various medical encounters documented in the medical records provided they led to the charges contained in the medical bill. This information can be used to automatically cross-reference the information contained within the medical records with various line items on the medical bill, therefore dramatically accelerating the process of recovery, while at the same time reducing the underlying costs.
Until now, the prohibitive costs associated with these kinds of audits have meant only large bills are investigated in this manner. By accelerating the speed of processing and reducing the associated costs, advanced technologies like intelligent text analytics and AI are broadening the possible scope of such investigations with the goal of maximizing revenue recovery.
About The Author
David Bayer is Vice President, Compreno Group at ABBYY. He has nearly two decades of experience across multiple domains, including content management, search, eLearning and eDiscovery.