Transforming Healthcare Through Big Data
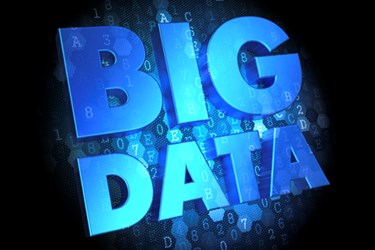
By Carol McDonald, Solution Architect, MapR Technologies
Healthcare has entered an era of major data transformation spurred by the use of advanced analytics and Big Data technologies. The catalyst for this transformation includes both the move toward evidence-based medicine and value-based payments.
These new approaches raise significant challenges. Implementing evidence-based medicine demands access to the most recent research and all available clinical data from a multitude of sources while factoring in advanced analytics to improve patient care and outcomes. The shift toward value-based payments requires significant improvements in reporting, claims processing, data management, and process automation.
An estimated 75 percent of healthcare data generated today is from unstructured sources such as digital devices, emails, clinical notes, laboratory tests, imaging, telematics, and third party sources. Many organizations are finding traditional, relational database technologies can handle neither this volume of data nor its unstructured nature. New technologies and thinking are needed to fully realize the healthcare data revolution.
This is where Big Data and advanced analytics come in.
Big Data Trends In Healthcare
- Reducing Fraud, Waste, And Abuse: Fraud, waste, and abuse contribute to spiraling healthcare costs in the U.S., but Big Data analytics is changing this. Using predictive analytics, the Centers for Medicare and Medicaid Services (CMS) prevented more than $210.7 million in healthcare fraud in one year which many believe is just a fraction of possible savings.
To identify fraud and abuse, insurers need the ability to analyze large unstructured datasets of historical claims using machine-learning algorithms to detect anomalies and patterns. By analyzing patient records and billing, healthcare organizations can detect anomalies such as the submission of duplicate claims, treatments that are not medically necessary, or providers who administer a higher rate of tests.
One major healthcare provider leveraged a data lake approach to aggregate massive volumes of data as a data hub for various departments, including fraud prevention. As a result, the provider is able to capture an incremental 20 percent of fraud, waste, and abuse in its claims department.
CMS uses predictive analytics to assign risk scores to specific claims and providers, to identify billing patterns, and claim aberrancies difficult to detect by older methods. Rules-based models flag certain charges automatically, such as an ID card that has been marked as lost or stolen. Anomaly models raise suspicion based on factors that seem improbable such as a doctor billing for the treatment of 50 or more patients in a day. Predictive models compare charges against a fraud profile and raise suspicion. Graph models raise suspicion based on the relations of a provider as fraudulent billers are often organized in tight networks. - Predictive Analytics To Improve Outcomes: The Health Information Technology for Economic and Clinical Health (HITECH) Act of 2009 accelerated the adoption of EHRs through a $30 billion federal grant. HITECH also provided incentives for the “meaningful use” of EHRs. Meaningful use includes the sharing of patient information between healthcare providers, patients, and insurance companies to improve patient outcomes and lower costs. As a result, the volume and detail of patient data is exploding.
With access to massive amounts of structured and unstructured patient data across a wide range of data sources, predictive analytics can aid in diagnosing patient conditions, match treatment with best outcomes, and predict patients at risk for disease or hospital readmission.
Predictive modeling is helping with the early detection of problems such as sepsis. Sepsis, an extreme and life-threatening autoimmune response to infection that can lead to tissue damage, organ failure, and even death, is often difficult to predict and diagnose as it mimics other conditions. The Agency for Healthcare Research and Quality identifies sepsis as the most expensive condition treated in U.S. hospitals, costing more than $20 billion in 2011. According to the CDC, the average hospital stay for sepsis costs twice that of other diagnoses and the mortality rate for patients with septic shock, the most severe stage of sepsis, is nearly 50 percent. Predictive analytics using real-time EHR data such as heart rate, respiratory rate, temperature, and white blood cell count can identify sepsis, which results in earlier diagnosis, faster treatment, significantly reduced mortality rates, and a lower overall cost of care.
The availability of millions of patient records means predictive analytics can find not only similar symptoms but also patients who are the same age, gender, ethnicity, and even have a similar response to a specific medication. By analyzing vast data sets across different systems, Big Data and predictive analytics are informing healthcare decisions and providing real value for companies.
- The Healthcare Internet Of Things:
The Internet of Things (IoT) refers to the rapidly increasing number of smart, interconnected devices and sensors that share data across the internet. In healthcare, these devices monitor almost every type of patient behavior from blood pressure and electrocardiogram monitors that capture heart function to Band-Aid like biosensors that detect the early stages of sepsis.
Though both practitioners and patients benefit from these interconnected devices, the tidal wave of data can be overwhelming. Many of these measurements require a follow-up visit with a physician. Smarter monitoring devices that communicate with other patient devices could greatly refine this process, lessening the need for direct physician intervention and replacing it with a phone call from a nurse. Other smart devices already in place can detect if medicines are being taken regularly at home from smart dispensers. If medications are not being taken, the device can initiate contact from healthcare providers to ensure patients are compliant with their medication. This real-time monitoring can have a significant impact on managing of chronic diseases such as asthma and diabetes.
Spending on healthcare IoT could top $120 billion in just four years. The possibilities to lower costs and improve patient care are almost limitless. Big Data analytics will play a major role in this as most of the data created by the healthcare IoT is unstructured.
It isn’t just the unstructured nature of most healthcare data that matches up well with Big Data analytics. It is also the sheer enormity of the data volumes from these and other sources, which would easily overwhelm traditional analytics platforms.
Lowering Costs And Improving Patient Outcomes
Improving patient outcomes at the same or even lower cost is an extraordinarily tall order for any healthcare provider. Full-scale digital transformation is the key to reaching this goal. Next generation technologies will achieve this through data convergence, stream processing, and application agility.
Data convergence is the ability to handle massive amounts of data from disparate sources, from structured historical data in data silos to unstructured data from internet connected devices. Convergence combines the immediacy of operational applications with the insights of analytical workloads, which historically have been separate.
Stream processing is the ability to analyze streaming data off the wire. Data that in the past would be aggregated and queried later is now being analyzed immediately in real time. This ability to make care decisions based on real time data has numerous life-saving applications.
Finally, next generation applications need to be agile to meet the demands of developers, data scientists, business analysts, and executives so that the healthcare industry can deliver improved patient outcomes at a lower cost.
About The Author
Carol has extensive experience as a developer and architect building complex, mission-critical applications in the Banking, Health Insurance, and Telecom industries. As a Java Technology Evangelist at Sun Microsystems, Carol traveled all over the world speaking at Sun Tech Days, JUGs, companies, and conferences. She is a recognized speaker in Java communities.