The Data Scientist Dilemma – 6 Signs You Need Self-Service Data Prep
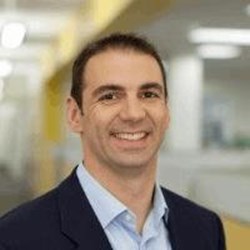
By Frank Moreno, VP of Product Marketing, Datawatch
When it comes down to it, what industry has a greater need to transform data into actionable intelligence than healthcare? From clinical analysis for decision making about patient treatment and preventative care, to operational analysis for revenue cycle management and patient experience, making data-driven decisions in healthcare is high stakes. And with ever-increasing volumes of data to wade through, the healthcare market’s demand for data scientists is skyrocketing, as it is in other industries (you may have heard that the role of the data scientist was recently dubbed the best job in America by Glassdoor).
Yet, the role of the data scientist is not without its share of challenges. Patient information and other data are rarely analysis-ready because it comes from multiple, disparate sources and in varying formats. Data scientists must also determine how to work with their IT counterparts to request, access, and utilize information while remaining in compliance with industry and regulatory requirements. Compounding the problem is the fact healthcare executives can make unrealistic, time-sensitive requests for reporting, visualizations, and analysis. (Does this sound familiar?)
This all leads to what we call the “data scientist dilemma.” To meet their organization’s demands and provide accurate reporting, data scientists may have to:
- work days, nights, weekends
- limit analysis to only the data they can easily access
- manually rekey data
- cut, copy, and paste data into Excel
- figure out how to combine disparate data sources
According to the 2016 Data Science Report released by Crowdflower, 76 percent of data scientists view data preparation (prep) as the least enjoyable part of their work, even though data prep accounts for 80 percent of the work they do. It’s bad enough data prep is incredibly laborious; but, what’s worse is data scientists are forced to compromise analytics.
However, there is a cure for all of these ailments — self-service data prep. With data prep tools, healthcare organizations can boost data scientist productivity, speed time-to-analysis, and get better insights for better outcomes, as well as empower anyone to be a data scientist. Best of all, self-service data prep provides an added benefit by allowing data scientists to use pre-existing reports and combine disparate file types so that the number of data set and BI report requests submitted to the IT department will decline. It brings a sense of well-being across the healthcare organization.
The “data scientist dilemma” is one sign your organization needs data prep; here are six more to consider:
1.More time is spent collecting and preparing data from multiple EMRs and different hospital departments than analyzing it.
2.There’s a heavy reliance on the IT department for data access and specialized reporting.
3.Business decisions are being made based on outdated or incomplete data.
4.Errors are becoming too frequent because volumes of data in varying formats are being manually rekeyed from disparate sources — unstructured (paper records, radiology images), structured (PDFs, patient databases, EMRs, billing statements), and semi-structured (JSON, XML, online charts).
5.The cost of a self-service data prep solution is less than the combined salaries of the team currently managing the data — up to $22,000 per year per analyst.
6.Data governance is an ongoing struggle, especially given HIPAA regulations for masking patient-sensitive information.
If your organization is indeed suffering from these symptoms, it’s not alone. With self-service data prep, you can change the prognosis, just like some of your peers have.
- Southeastern Med, a healthcare center and community hospital in Cambridge, Ohio, needed to achieve broader and deeper business intelligence reporting by tapping data from a wider variety of sources. With a self-service data prep tool, the team is able to access, blend and cleanse data, then feed it into an advanced analytics platform. As a result, Southeastern Med is able to more efficiently and effectively track and report on hospital infections, improve physician performance and identify treatment trends.
- Cancer Centers of the Carolinas (CCC), a community-based physician-owned practice, grappled with data reconciliation, especially for revenue cycle management where the business team dealt with claim submissions and denied claims. Using self-service data prep for this operational use case, the team is able to accurately predict future trends and have information on what outstanding charges need to be billed to insurance companies. The clean data has significantly decreased the number of denial rates and payables.
- Piedmont Henry Hospital, a non-profit community hospital in the Atlanta area, sought to provide timely, accurate information to all necessary hospital staff to improve the patient experience, increase revenue and meet regulatory requirements. Using repeatable data models quickly built with a self-service data prep tool, the hospital was able to combine structured, unstructured and semi-structured data to create a comprehensive new report methodology that included report routing and distribution — all while maintaining HIPAA compliance.
Data scientists shouldn’t have to compromise on information quality — ever. Self-service data prep eliminates the data scientist dilemma, while enabling organizations to recoup precious hours that can instead be devoted to analysis that will inform critical decision making about medical operations and patient care.
About The Author
Frank Moreno is VP of product marketing at Datawatch, a provider of data preparation software for analytics and operational use cases. He has worked in the enterprise and infrastructure software industry for more than 20 years, serving in various senior-level product marketing and marketing communications roles at companies such as PeopleFluent, Kronos, Cadec Global, and Empirix.