Tech Companies, The FDA And Big Data Where They Are Headed
By Gal Salomon, CLEW Medical
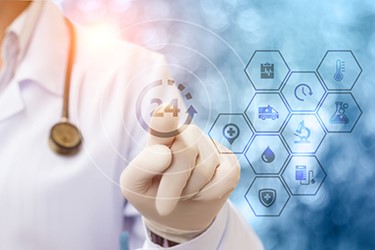
There is a plethora of data available to doctors and hospitals today, which is collected from medical devices, instruments and monitors, in settings from doctors' offices to hospital wards to emergency trauma centers. In addition, personal devices, from Apple Watches to Fitbits and everything in between, are collecting steady streams of information on a variety of metrics, from heartbeat to movement and activity data to calories consumed.
It's all part of the big data revolution, and if used properly, all of this data could provide medical personnel with important insights on patients' health. But as often happens, technology has outpaced regulation – and it is only now that regulators, namely the FDA, are beginning to decide how big data can be used in diagnosis and evaluation of patient conditions, and especially patient outcomes.
Big data, and the analysis of data, has become very important in many industries. In manufacturing, predictive analytics lets managers know when machines need specific kinds of maintenance in order to ensure they keep working; in retail, predictive analytics helps businesses know what sales are expected, how much product to order, etc. These are just a few of many examples.
Big data and predictive analytics could also be used in medical settings. Using profiles of patients suffering from specific diseases, and taking into consideration their medical histories, current conditions, and multiple other factors, systems could analyze a patient's real-time condition and predict likely outcomes for doctors. The data that is collected and analyzed is compared to historical data or profiles of patients or cases that match the patient being examined.
For example, if a patient suffering from a specific form of cancer displays certain physiological conditions, doctors could use the data derived from devices, the patient's profile, etc. and check it against the experience of others in order to determine what those physiological conditions mean in the context of treatment and aggressiveness of the cancer.
Medical personnel are able to get a clear picture of what is wrong with a patient, and what needs to be done to help them. It's a much more logical and efficient way to allocate precious resources in hospitals and other treatment venues. Predictive analytics has proven itself in other industries, and there is no reason it shouldn't prove itself in the medical industry - especially in the intensive care unit, where every moment is of the utmost importance. Analytics could prove to be an important method of saving lives.
So what's the holdup? Regulators in the medical industry are more conservative than in other areas – understandably so. With human lives on the line, regulators seek to be as careful as possible when approving new treatments, devices, or medical systems. And while data analytics has proven its chops in other industries, regulators want to see proof of its efficacy in medical treatment.
However, the issue is that analytics, including the methods used and the data analyzed, varies on a case by case basis. Two patients with ostensibly the same metrics, history, and symptoms – at least from what is evident on the surface – could find that they are assigned two completely different modes of treatment after their cases are analyzed by big data systems. Pinpointing the exact factor that would account for that difference in advance of the analysis could be very difficult, if not impossible, given the huge number of variables.
This goes against what medical regulators generally look for when approving new procedures, devices, or systems. Medical treatment – and approval for medical treatment by the FDA – is generally evidence-based, having to prove why this specific treatment is warranted. As the evidence is different in each case, the FDA has until now been reticent to accept predictive analytics as a criteria for selecting treatment. But progress cannot be stopped. Recently, the FDA has issued very preliminary guidance on how to use big data analytics and machine learning.
With that, the path to regulatory compliance is still unclear. The FDA is seeking to clarify some aspects of big data analytics, such as how to avoid bias and causality leakage in AI, how to ensure accuracy in data processing, how to prevent changes in units (i.e. pounds to kilos, percents 99 vs. proportions 0.99), what methods can be used to validate an analytics-based decision to ensure it makes sense, and other issues.
For those clarifications, the FDA will be relying on companies that are building data analytics for use in medical settings, to design the studies that will validate their approach. The proper scientific approach is to build a corpus of studies showing the efficacy of data analysis in specific conditions/diseases, and work from there. With the studies, the FDA could examine the general method applied, and develop rules on how big data can be used in a medical setting. The approval could be authorized for specific scenarios as well, such as in the ICU or emergency room, where patients need to be stabilized as quickly as possible.
A good example of the process in action is the FDA's recent approval of a system for leveraging data within a hospital’s existing electronic health record (EHR) to quantify and visualize patient deterioration, risk and improvement in real time. The research in those articles led the FDA to issue the regulations on the system – and that is the process we hope to see in the application of big data analytics to treatment and medical outcome predictive systems. Approval of those systems is, we believe, inevitable – there is so much technology and advances in data analytics that it cannot be ignored. The more data analytics development firms help the FDA with the process, the sooner that approval will come.
About The Author
Gal Salomon is CEO of CLEW Medical.