Privacy Analytics Enables Richer Insight Into Anonymized Clinical Data

By Christine Kern, contributing writer
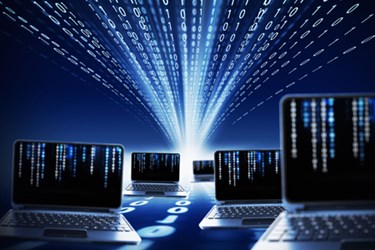
Gaining Optimal Analytic Utility from Anonymized Data
Currently, healthcare systems are in midst of extensive reform to reduce costs and increase efficiency in approaches to primary delivery. In Canada, the Canadian Working Group for Primary Healthcare Improvement has recommended performance reporting be a strategic priority for the primary healthcare system. This requires rich repositories of data and analysis that are often highly personalized and limit aggregate analysis of populations.
A critical source of primary care and delivery data is electronic medical records (EMR). EMR data not only enables highly detailed performance reporting, but also allows for comprehensive, near on demand analysis by automating data collection.
Recently, a leading healthcare analytics firm was faced with the daunting task of anonymizing more than five years of clinical, prescription, laboratory, scheduling, and billing data for a database of more than 535,000 patients in the province of Ontario. The complexity of the project was challenging, given that the EMR vendor collected data from 5,820 healthcare providers and 2,664 clinics. Adding to this complexity was the relative size of the data set, which had 820 columns and 75 tables.
To complete the task, they chose to apply Privacy Analytics software, PARAT to a complex longitudinal data set and leverage its insights for on-going and on-demand analytics. What resulted was high-quality anonymized data for post-marketing and public health surveillance, prescription and health service analyses.
“We worked very closely with our healthcare analytics partner to create a repeatable, yet scalable approach to de-identification,” said Luk Arbuckle, Director of Analytics for Privacy Analytics. “We had two key objectives: 1) ensure we provided de-identified data set that could be refreshed quarterly; and 2) demonstrate that the analytic quality of the original and de-identified data sets were the same.
“As a result of our analysis, we could confidently inform the healthcare analytic vendor that they could gain an optimal range of analytic utility and value. “This would allow for post-marketing and public health surveillance.”
The study found that:
- Most analyses performed on clinical database use descriptive statistics and tabulations.
- Anonymization meets the requirements of these techniques, while maintaining the essential analytic utility of the original data.
- Data evaluation should be statistical as opposed to deterministic, comparing a before and after approach of an anonymized data set.
- Anonymization allowed this vendor to fully leverage their data for a secondary purpose within a reasonable range of optimal utility and value.
The highlights of the study are available in a webinar, here.