4 Obstacles Blocking Business Intelligence In Healthcare
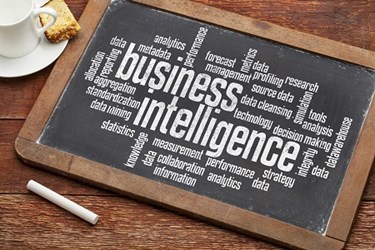
By Abby Adams, Senior Business Analyst, Itransition Group
Among all other industries, healthcare is no doubt the most hyper-personalized. However, while care depends on the personal relationship between patient and physician, it is also inextricably bound to technology. From EHRs to a less adopted CRM, every solution generates and processes tons of complex data. Big Data.
Big Data follows healthcare by default, and it hides plenty of hints and clues for balancing costs, improving care, complying with industry standards, and defining the strategy for further growth.
The challenge is creating a working business intelligence (BI) strategy that brings real, valuable insights to base decisions on. According to Gartner’s research, the lack of an effective BI strategy appears to be a major flaw in attempts to improve business operations in healthcare.
But what is holding providers back from setting up a flawless BI strategy? Let’s figure it out.
High Data Sensitivity
Healthcare organizations deal not only with patients’ financial information, but also with sensitive patient and clinical data regulated by the strict HIPAA rules which require extra security when handling information. When it comes to data analytics, caregivers need to think through the strategy of managing access to private information, distributing roles, and making sure they keep data safe across all sources and data warehouses.
Difficulties Of Data Access
Large healthcare organizations, such as ACOs, can have 15 or more EHR systems in use simultaneously. Some of these systems can be built in-house, whereas others may be hosted by one of the EHR vendors. As every EHR has its own backend database, pulling information from all of them actually takes knowing each database’s specifics. Moreover, it may take not only building a single ETL algorithm to pull data, but also writing additional code to make data storages communicate with each other.
In this case, caregivers would probably have to turn to either an EHR conversion company to bring disparate data together and then leave it to the provider’s BI team, or leave the whole process of joining information sources to a healthcare-oriented business intelligence company. Either way, this effort will be handled by savvy businesses which know most EHR systems on the market, as well as their unique attributes.
It is also important to remember not every EHR vendor wants to share their source code or integrate with third-party analytic systems as their policy may not allow sharing data and/or extracting it from the system. In some rare cases, vendors might not even be willing to provide access to source code for a charge.
While their motives are questionable, and we hope no caregiver will encounter such an unpleasant situation, there is always plan B. To make improvements with low risks, it is essential to make clinical and patient data available to the analytical and reporting environment. So organizations need to think through their line of behavior, consult with their legal team, and find appropriate levers in case these problems occur.
Data Quality
Accessed data successfully? Congratulations, yet the resulting quality fail to meet your expectations. And there’s a whole range of reasons why.
As previously mentioned, healthcare data comes from different sources —EHR, ADT, ERP, and more. It also travels to different departments such as radiology, cardiology, pharmacy, and others. Moreover, data is generated in multiple shapes and forms, such as videos, text, numeric, images, multimedia, and PGHD —patient-generated health data that comes from smart watches, wearables, and mHealth applications. Radiology uses pictures; labs go with texts, tables, and numbers; old medical records can still exist on paper exclusively.
It is also the case when the same patient information piece is presented differently across systems and formats. For example, a patient’s emphysema looks like an image in the medical record, but becomes an ICD-10 code J43.9 in the claim.
Now, as adopting an EHR is a costly and mostly long-term process (it can take about six months), providers stick with a system rather than rip and replace, and those first-generation EHRs weren’t that advanced. Practically, their security protocols were too weak so clinical data was stored non-discretely within documents, which made this information inapplicable for reporting.
Also, when all collected data finally gets processed, a caregiver can find a lot of data in their analytical system hasn’t been stored as structured, codified values. Instead, the BI team faces tons of unstructured text information. Mapping these values and structuring them across systems to extract valuable insights and get reporting possibilities is a resource-exhausting and time-intensive process.
An effective BI strategy implies all tech-run approaches used by providers will help them turn bad data into good data in the first place. Algorithmic data mapping and NLP (natural language processing) techniques are to be demanded from any data scientists whom caregivers turn to, both in-house and elsewhere. While transforming information is not an easiest task to perform, without quality data it’s impossible to achieve any visible results.
Data Inconsistency
Different health specialists, such as physicians, surgeons, nurses, and others, input data in many ways. EHRs and other provider systems allow storing similar data types in multiple areas within each solution. When caregivers want to obtain this information, a challenge occurs.
The flexibility of entering information shows its ugly, inconsistent side when it comes to data pulling and reporting. The BI strategy needs to consider the need of removing duplicate data and flawlessly synchronize disparate information parts belonging to the same patient profile.
Information across multiple systems (EHR, financial, claims, and operational) at large healthcare organizations differs a lot, thus bridging data is challenging too. Each system will identify the same data elements differently, which hinders understanding and consistency. Likewise, they will store and handle data parts that may or may not have an equivalent element within other solutions.
Still, providers need to bring all this data together and make it work in order to harness BI and use it to its full potential. While most systems offer extraction options, they send data in many various formats. It can also be information received from external and internal sources. So the ETL process and other solutions dedicated to aggregating information and sending it into a general data warehouse should be robust enough to deal with all possible formats, find common points in data, make it consistent, and result in a consolidated view offering only meaningful findings.
Overcoming The Challenges
Now we understand a number of pitfalls that await those who strive for improvements in healthcare. However, the challenge itself isn’t an issue. Knowing about possible ways, solutions, and approaches to overcome any gap is just a part of the process. Whether it is an in-house BI team or a dedicated business intelligence company, they should be a professional and trusted partner, because this partnership has a potential to become a life-long marriage due to shifts in the pay-for-performance reality.
Value-based care is just kick starting its way to a more patient-centric and clinically effective environment, thus healthcare data analytics, BI, and machine learning are here to stay. Why is that?
The bar is rising quickly, new performance metrics appear, care quality becomes measurable, patient outcomes start to go way further than admission, readmission, and mortality rates. So it’s not only about achieving, it’s about staying relevant and also unlocking new opportunities such as:
- reforming the patient care delivery
- identifying areas for internal performance improvement
- improving patients’ health outcomes
- seamlessly reporting to government and commercial payers
- managing financials to balance costs and more