NLP, AI, And Machine Learning: Complementary Technologies For Advancing Patient Care
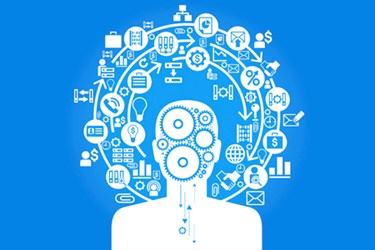
By Simon Beaulah, Senior Director of Healthcare, Linguamatics
For the past few years, artificial intelligence (AI) — especially natural language processing (NLP) and machine learning — has been a hot topic in healthcare as vendors, researchers, and providers consider ways to leverage technology to transform medical research and clinical care. As the volume of electronic clinical information continues to grow, AI and machine learning are poised to revolutionize pattern identification, decision-making, and outcome prediction.
Machine learning can help to solve complex problems by analyzing existing data or past experiences. For example, by analyzing past clinical outcomes from various treatments, machine learning could potentially predict future health outcomes based on specific treatments. Effective machine learning however, requires access to good quality data in a structured format in order to train the algorithms used for analysis.
In healthcare, the volume of accessible clinical data has skyrocketed in recent years, thanks to the near-universal adoption of EHRs. The wealth of clinical data has helped drive significant insights into the health of populations. However, because about 80 percent of the data stored in a typical EHR exists in an unstructured format, efforts to analyze the health of individuals have been hampered.
Fortunately, the use of AI techniques like NLP can effectively speed the extraction of critical information from unstructured clinical text. NLP helps to swiftly interpret the structure and meaning of the language stored within unstructured free text and translate it into key concepts in a structured format — providing a more detailed, comprehensive view of the data. Machine learning can then be applied over the entire data set to assess patterns and trends, and to identify opportunities to reduce costs and improve population health.
What’s The Machine Learning/NLP Connection?
NLP software helps make more data usable for machine learning applications; in some cases, machine learning also enhances the capabilities of NLP systems. For example, machine learning can aid NLP systems by identifying language structure to accurately label a word as a noun versus a verb. While machine learning can enhance the functionality of NLP applications, the addition of NLP to machine learning applications makes both semi-structured and unstructured data easily accessible without the need for labor-intensive processes, such as the manual extraction of individual patient charts to capture discrete key concepts.
Which Type Of NLP Is Best?
NLP facilitates the extraction of clinical data from free text within EHRs and other clinical documents so that machine learning analytics can be applied. But which type of NLP software is best to achieve this? The answer to that question depends on the user’s needs.
Statistical NLP systems require real-world example data to infer patterns in data. The examples may come from dictionaries or ontologies, and may require laborious manual annotation by a clinician. Meanwhile, most rule-based NLP systems require a specialist to define the types of language rules or patterns that represent healthcare concepts, instead of inferring the presence of the concepts from labeled data. This approach may make the rules more accurate and specific, but the rules may be harder to maintain and be limited to those patterns that the specialist has thought of.
A third and more recent alternative is data-driven, rule-based NLP, or agile NLP-text mining. This method makes it easier to create, edit, and reuse patterns, even when the user is not a specialist in NLP. Though this method can make use of dictionaries and ontologies, it does not require labeled data because it is not primarily a statistical NLP system. Ultimately an agile NLP-text mining system can produce data, or features, to be used in downstream machine learning models. For example, key phrases and attributes, such as life style choices and social determinants of health, can be extracted from unstructured text in clinical reports with significantly reduced time compared to manual chart extraction. This enables faster, comprehensive, and consistent input to accelerate the development of new machine learning algorithms.
Leveraging The Use Of NLP In Machine Learning Projects
NLP, as part of the AI trend, can provide access to clinical data trapped as unstructured text and make it available to advance the development of machine learning algorithms. These complimentary technologies can deliver a powerful solution to help healthcare providers and researchers understand patient needs and seek advancements in the delivery of care.
About The Author
Simon Beaulah is Linguamatics’ senior director of healthcare and is responsible for the company’s healthcare products and solutions, including applications for clinical risk models, population health and medical research.