ML For Healthcare As A Requisite To Responsible Patient Care
By Chris Lehman, SADA Systems
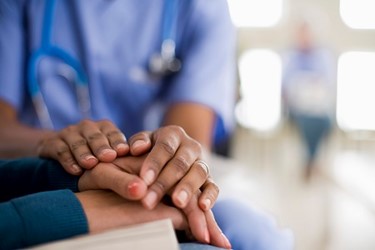
Big data has long since arrived in the healthcare provider industry. We live in a world where remote patient monitoring devices can generate hundreds of blood pressure readings per day, where every supply is tracked down to a strip of gauze, and millions of radiology images may reside within a single EHR server. But along with these piles of data come clinicians and analysts charged with sifting through them, and the herculean task of providing actionable insight. Thankfully, machine learning technology has matured in tandem with infrastructure that handles Big Data to the point where machine learning is no longer just a buzzword. In this age, hospitals not only have the ability to implement machine learning in practice, but an urgent responsibility to do so in order to provide modern patient care.
Care Of The Future: Human Judgement With Machine-Supported Decision Making
The future of patient care will draw from human strengths such as layered reasoning, creativity and empathy while merging machine strengths of efficiency, speed, and unbiased discernment. In practice, one can picture a clinician using live visual and auditory input from a patient encounter while referencing machine classifications from vital stats, past bloodwork, or EKG readings run against a global backdrop of comparison data. Machine learning algorithms can provide real time insight from Big Data, an issue that humans are not particularly suited to face.
Until recently, the healthcare provider industry has lagged to adopt machine learning for valid practical, technical and organizational reasons. Many providers are still maturing into modern EHR systems or cloud workflows, while others struggle to find development talent to implement such complex technology. Many recent advancements in Platform as a Service offerings have since mitigated these concerns. Google Cloud, for example, now offers pre-built machine learning libraries and ready-to-use APIs that do not require a team of PhDs to get up and running. Whether the application is Natural Language Processing to derive clinical insights from a patient’s past clinical notes, or image recognition models that are equally capable of deciphering doctors’ scrawl as they are poring over CT scans, providers will find much of the heavy lifting complete. Developer-friendly documentation now exists where 100-page dissertations used to live.
Breaking Down Barriers To Incorporating Machine Learning In Practice
In spite of these advances, providers will still come across technical and organizational obstacles. For a vast array of machine learning initiatives, the most time-intensive part of the buildout is the aggregation and massaging of data for feeding into a model. Many providers must pull across legacy EHRs and link together a variety of on-premises and cloud systems. My advice from experience is to start small. Begin with a clearly defined business objective involving a narrow list of stakeholders and rely on a single data source when possible. For a healthcare system in practice, this may look like using data for providers that share a single EHR (and reaching back for discharges spanning several years) instead of trying to extract data across EHR systems for the current year. It’s also possible to start small with a few thousand records of data to create a proof-of-concept. One can always bring in new data fields to further tune a model (this term is called Feature Engineering) or add more data at a later point in time.
Pick A Problem To Solve Before Choosing Your Solution
For many hospital administrators, it’s difficult to come up with a clearly stated business case for machine learning that all can buy into. Much of machine learning boils down to predicting classifications or patterns from large sets of data. Using supervised learning techniques, models are trained from input data and pre-labeled output data. For example, the input data may be thousands of images of skin lesions, and the labeled output might be “cancerous” or “benign.” This method is particularly attractive to clinical decision making. As electronic health records mature, many hospitals are sitting on treasure troves of clinical input data and labeled outcome data - readmissions, patient satisfaction, or survival - to match.
On the flipside, unsupervised learning models used unlabeled input data to suggest patterns in data that could lead to clinical and financial insight. By feeding in demographics, financial and ICD-10 diagnosis data for patient encounters, an unsupervised learning model might group patients across seemingly distinct clinical profiles. Coupled with human analysis, these groups may then present different patterns of resource usage, readmission rates, or likelihood for denials.
By generally understanding the different approaches to machine learning, administrators are more capable of working around data limitations specific to the business case.
Machine Learning Projects Require Measurement, Iteration And Patience
Regardless of method chosen, development will always be an iterative process of trial and error. In practice, how are end users supposed to trust a prediction suggested by a machine learning model without seeing inside the black box? By comparing machine learning predictions to verified outcomes, a model can self assess its precision (how many times was the classification correct?) and recall (how much of the total population did I catch?). As developers further tune the model, and as the model trains on more examples, these metrics will increase until they are reliable to support human decision making. Administrators can set KPI gates around model accuracy before rolling out into a live environment.
Like any investment in new technology, recognize that change will not come overnight. Set a reasonable time frame for finding the right internal or external talent to build machine learning workflows, understand the business needs, deploy into production, and iterate the model to provide useful results.
One thing is for certain: the time for providers to invest in machine learning technology has arrived. Soon it will not be new or advanced technology, it will simply become ubiquitous technology in the way we view EHRs today.
About The Author
Chris Lehman is Head of Engineering for Google Cloud at SADA Systems.