Managing Complexity In Clinical Quality Measurement
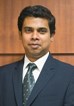
By Dennis Swarup, Vice President and Head of Healthcare BI & Analytics, CitiusTech
Healthcare industry dynamics are quickly changing due to greater focus on population health management and accountability of care through federal/commercial incentive programs (MU, PQRS, IQR, P4P) and payment reforms (shared savings, value-based purchasing). Clinical quality measures (CQM) are at the heart of these recent reforms and becoming increasingly important for healthcare organizations that seek to:
- Leverage clinical data to improve quality of care,
- Comply with federal/state regulatory reporting requirements,
- Meet performance benchmarks associated with P4P and value-based payment programs.
Healthcare organizations need to ensure they are able to respond to escalating CQM requirements, as well as support the scale and diversity of clinical information across various specialities and care settings.
Supporting CQM requires considerable effort in interpreting measure definitions, defining decision trees, implementing clinical rules, testing possible scenarios, and training and supporting end users. Managing complexity of this nature and scope is not easy, and successful organizations will need to focus on multiple dimensions.
Getting an aggregated, reconciled and standardized view of clinical information
Many healthcare organizations have had limited success delivering a comprehensive view of patient information, often relying on manual processes to reconcile aggregated data, calculate measures and generate reports.
Sole reliance upon an EHR poses significant challenges. Members of the College of Healthcare Information Management Executives (CHIME), for instance, recently told CMS officials that quality measures through EHRs are “extremely time intensive and difficult.” The statement from CHIME reiterates the fact that “data used by abstractors are often found in dictated reports or free form progress notes, not as structured data in the electronic health record,” and that “one cannot extract all the data needed on every patient to create accurate quality metrics.”
This is largely due to a wide array of disintegrated clinical and financial applications that organizations have deployed over the years to meet the needs of specific department and end-user communities (e.g., cardiology, oncology, pathology). This multiplicity has been compounded by the uptick in industry mergers and acquisitions.
A typical integrated delivery network (IDN) includes more than one hospital with varying specialties, for example, with each deploying best-of-breed clinical and financial applications. To get a centralized and consistent longitudinal view of clinical quality performance for inpatient services, it is imperative for the IDN to aggregate data from the various hospitals, reconcile the information at the patient level, and standardize this reconciled data to ensure uniformity of interpretation.
The problem becomes manifold when extended to additional entities like physician practices, ambulatory surgery centers and health plans. It becomes exponentially more difficult to manage clinical quality performance for individual patients, as well as at the broader population level across the continuum of care.
Capturing clinical data in discrete, referenceable, and interoperable formats
Clinical data includes an extensive set of information types, ranging from family history, social habits, diagnostic tests, clinician assessments, medical history, care interventions and treatment plans. As hospitals introduce new workflows and technology capabilities to support increasing numbers of CQM, they face numerous challenges around discrete data capture, data quality and standardization, including accuracy/integrity, searchability, completeness, quality, redundancy and consistency with interoperability standards such as SNOMED, RXNORM and LOINC.
By using a terminology standard (such as SNOMED), organizations need to build standardized clinical concepts into applications such as EHRs. This allows data to be captured electronically and shared across diverse applications with standardized reference and uniform interpretation to provide a unified view of quality performance across the ecosystem. Organizations must also periodically update the support concepts to comply with the evolving vocabulary standards and constituents.
Interpreting clinical guidelines for effective population health management
The effectiveness of population care measures depends greatly on the organization’s ability to accurately define risk-stratified patient cohorts and applicable care measures to generate actionable insight to manage outcomes.
Healthcare organizations face the challenge of interpreting an extensive and complex set of evidence-based clinical guidelines, as well as accounting for justifiable exceptions, in order to include the right patients in a cohort created for specific targeted initiatives such as disease or wellness management.
To segregate a simple cohort of diabetic patients, for example, the clinical analyst needs not only to include patients with an active diagnosis of diabetes, but also patients with medications indicative of diabetes. He or she likewise must exclude patients with gestational or steroid-induced diabetes.
Leveraging BI/analytics to implement, track, manage and report CQMs
An integrated approach to BI/analytics can help organizations manage data and support clinical measures in a scalable manner across multiple initiatives from a centralized repository. Key drivers for the use of BI/analytics to manage CQMs include the ability to:
- Aggregate data from multiple source systems and apply the necessary transformation, terminology mapping and reconciliation rules to ensure data consistency.
- Review quality measure calculation granularly, and identify data abstraction and requirements to ensure integrity and completeness of reported data.
- Define patient cohorts based on clinical conditions and/or risk profiles, and track care measures against cohorts.
- Publish and distribute performance metrics/reports to improve transparency and influence end-user behaviour.
- Benchmark performance at various levels of the organizational hierarchy, as well as national/regional indices, and generate regulatory reports in prescribed electronic formats (XML, QRDA, etc.)
To manage the complexity of CQM effectively, organizations need to build a holistic roadmap to bring clinical and financial information together, create processes to achieve high levels of data quality and standardization, and appropriately interpret and implement evidence-based clinical guidelines. Effectively leveraging BI/analytics tools such as those offered by Citius Tech to manage clinical quality, financial and operational performance is key for healthcare provider organizations to manage the shift in accountability and risk-sharing, and transition to newer payment models.
Dennis Swarup is Vice President and Head of Healthcare BI & Analytics at CitiusTech. He has extensive experience in technology consulting and development for Fortune 500 clients, around healthcare BI/analytics, clinical quality and performance management. Dennis holds a Bachelor’s degree in Technology and has a Master’s Degree in Information Systems.