Machine Learning Exposes The Secrets Behind Healthcare FWA
By Lalithya Yerramilli, SCIO Health Analytics
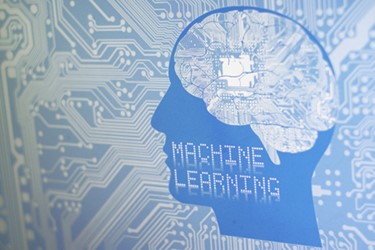
When most of us think of spies, the image that immediately springs to mind is someone cool, handsome or beautiful, and highly cultured. James Bond, of course, is the archetype of that image.
While that makes for entertaining movie-going, in real-life spies tend to be very plain, ordinary-looking individuals. Which makes sense.
A spy’s job is to blend in to a crowd so he/she can move about unnoticed, which is a very effective way to gather information. It’s kind of tough to blend in when you’re clearly the best-looking and most charismatic person in the room.
The same principle is true for bad actors looking to commit fraud, waste, and abuse (FWA) in healthcare. While in the movies they would be hatching all sorts of complex, nefarious schemes to create a big score, often involving very sophisticated technologies obtained on the dark web, the reality is much simpler. They just need to blend in.
Take people who want to obtain a large quantity of opioids, either for themselves or to sell. They’re not going to go to a single provider or pharmacy and try to get them all at once. That would be too obvious.
Instead, they will spread their requests across many providers and pharmacies. Obtaining 10 pills from 10 different sources is far less likely to gain attention than requesting 100 from one source.
That’s what makes uncovering FWA in healthcare so difficult. Most payers and providers don’t have the technology or human resources needed to gain a big-picture view of the enormous quantity of claims they receive each day. This fact makes it difficult to find subtle patterns indicating FWA. As a result, it’s easy for those who are careful in their approach to committing FWA to fly under the radar.
Since FWA has an estimated cost of anywhere from $80 billion to $272 billion each year in the U.S., however, it’s critical that payers and providers take action to prevent it. This is where machine learning, a subset of digital intelligence that combines data with human domain knowledge to create context around information, can be healthcare’s ultimate spy gadget.
Machine Learning Uncovers Patterns
In the spy game, cryptographers use pattern recognition technology (among other tools) to discover the secret messages within what appears to be gibberish. Machine learning in healthcare can do the same, parsing through massive amounts of data and hundreds of potential decision points to find subtle relationships and previously hidden patterns within seemingly unrelated events – pulling back the curtain those committing FWA were hiding behind.
Machine learning uses predictive analytics to build an initial set of models indicating what “normal” patterns are. It then looks for anomalies or occurrences that fall outside those norms. When one is discovered, it alerts human experts who use their experience to determine if further action is required. As data about the outcomes is added, the models are refined even further, helping the organization’s limited resources focus on activities that require attention while reducing false positives.
Here’s how that applies to the earlier opioid example. Payers and providers are often challenged to separate legitimate instances of patients/members seeing multiple providers and filling prescriptions at multiple pharmacies (as in the case of cancer patients with multiple co-morbidities) from patient/member drug-seeking behavior. It can be difficult to determine how many is too many.
Human experts might take months to sift through the volume of data required to develop rules around this issue that still may be incomplete. Machine learning, however, can uncover those patterns in hours, or a few days at most. This information can be used to confirm that a patient/member who goes to five providers and five pharmacies to get five prescriptions filled within a specified time frame is likely exhibiting drug-seeking behavior. The payer or provider can then address this behavior with reasonable certainty that it will yield positive results, including improving the patient’s/member’s health and preventing future FWA.
The more it is used within the organization, the more value machine learning can add – such as scoring the severity of variation rather than just pointing out a variation has occurred. This information helps investigators prioritize their efforts with greater precision, reducing FWA while delivering the best ROI for containing those costs.
Human Expertise Is Key
Taking advantage of machine learning in FWA doesn’t mean humans are no longer necessary. Quite the opposite. While machine learning can find the variables, human expertise is still required to determine how to address them.
Humans are also essential to improving the basic models, because there can be a huge difference between what is “normal” in an organization and what is correct. If a provider has never included the proper chronic condition code in a particular diagnosis, machine learning will pick that up as being acceptable. Humans will understand otherwise and create a framework to allow the algorithm to “train” itself to understand that when certain medications are prescribed, they should be associated with specific condition codes. If they are missing, the machine should point it out.
Overpayment of hospital bills is another area where the human factor is required. If an emergency department claim includes a charge for anesthesia where the procedure doesn’t ordinarily require it, a human may miss this discrepancy. Machine learning won’t. But since it may be justified in this instance, a human reviewer will need to decide if there was a legitimate exception in this case. If so, the algorithm will automatically amend itself to recognize this exception the next time, eliminating the cost and delay of a manual review.
Coming In From The Cold
Every dollar spent on healthcare FWA is a dollar that isn’t going into patient/member care. And since it is a multibillion-dollar problem in the U.S., the more that can be done to stop it cost-effectively the better it is for everyone – patients, providers, and payers.
Like spies, however, many instances of FWA are designed to be deliberately difficult to detect. Machine learning can help expose the subtle variations and patterns that indicate FWA is happening, helping healthcare organizations focus their anti-FWA efforts where they will pay the greatest dividends.
About The Author
Lalithya Yerramilli is Vice President of Analytics at SCIO Health Analytics. She has 15 years of experience in analytics in insurance, healthcare, and life sciences industries working with customer info-base, transactional, physician level, patient level, claims and longitudinal datasets.