How To Improve Healthcare Operational Efficiency Through Lean Principles And Predictive Analytics
By Sanjeev Agrawal, LeanTaaS
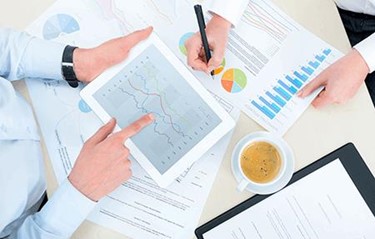
Operational dilemmas are experienced in all industries. Airlines, for example, are arguably more operationally complex, asset-intensive and regulated than hospitals, yet the best performers are doing a far better job than most hospitals at keeping costs low and make a decent profit while delivering what their customers expect. Southwest Airlines, for example, has figured out how to excel at the two operational things that matter most: Keep more planes in the sky more often, and fill each of them with more passengers and more often than anyone else. Similarly, winners in other complex, asset-intensive, service-based industries — Amazon, UPS and FedEx — have figured out how to over deliver on their promise while staying streamlined and cost-effective.
These companies have improved their operational efficiency through lean principles and predictive analytics. Below, I explain two reasons they are relevant to healthcare:
First, hospitals and hospital operations closely resemble airline and airport operations and transportation services. Each have many steps in their service operation (check-in, baggage, the security line, gates), high variability at each step (weather delays, congestion, mechanical issues), and multiple connected segments in the user journey — and all these operations involve people, not just machines. In mathematical terms, hospital operations, like airlines and transportation, consist of hundreds of mini-processes, each of which is more stochastic and less deterministic than, say, the steps in assembling a car.
Second, hospitals today face many of the same cost and revenue pressures that retail, transportation and airlines have faced for years. To remain viable, asset-intensive and service-based industries must streamline operations and do more with less, as Southwest, Amazon, FedEx and UPS have demonstrated. Healthcare providers can’t continue to try to spend their way out of trouble by investing in more infrastructure; instead, they must optimize the assets currently in place.
To become more streamlined and cost-effective, healthcare providers need to consistently make excellent operational decisions, just as the airline and package delivery industries have. Ultimately, providers need to establish an operational “air traffic control” for their hospitals — a centralized command-and-control capability that is predictive, learns continually, and uses optimization algorithms and artificial intelligence to deliver prescriptive recommendations throughout the system. Dozens of healthcare organizations are now streamlining operations by using platforms with the ability to mine and process large quantities of data to deliver recommendations to administrative and clinical end users.
Improving hospital operational efficiency through data science hinges on predictive analytics to improve planning and execution of key care-delivery processes, chief among them resource utilization (including infusion chairs, operating rooms, imaging equipment, and inpatient beds), staff schedules, and patient admittance and discharge. Done properly, providers see an increase in patient access (accommodation of more patients, sooner) and revenue, lower cost, increased asset utilization, and an improved patient experience. Some examples of areas where predictive analytics are improving hospital operations include increasing operating room (OR) utilization, reducing infusion center wait times, streamlining emergency department (ED) operations, ED to inpatient-bed transfer and accelerated discharge planning.
Increasing Operating Room Utilization
Operating rooms account for more than 60 percent of admissions and 65 percent of revenue at most hospitals. However, block-scheduling approaches fail at optimizing operating room time and in improving patient access, surgeon satisfaction, and care quality. Current methods — phone calls, faxes and emails — make block schedule changes cumbersome, error prone and slow. Using predictive analytics, mobile technologies and cloud computing, providers are mining utilization patterns to dramatically improve OR scheduling.
Reducing Infusion Center Wait Times
Infusion scheduling is an extremely complex mathematical problem. Let’s use a 35-chair infusion center that operates eight hours per day treating five types of appointments — one hour, two hours, 3-5 hours, 6-8 hours, and 9 or more hours — as an example. Assume four sets of patients can start their treatment at 10-minute intervals. That’s 256 possible start times or “slots” per day. The number of possible ways these patient appointments can be arranged is a number with over 100 zeros behind it. By comparison, if you were to use 1-gallon buckets to collect all the oceans’ water, the number of buckets you would need has “only” 40 zeros behind it.
Streamlining Emergency Department Operations
Anyone who has ever visited an emergency department knows it can be severely bottlenecked, whether because patients are waiting for lab results, the imaging department is backed up, or the department is understaffed. Analytics-driven software can determine the most efficient order of ED activities, dramatically reducing patient wait times. When a new patient needs an X-ray and a blood draw, knowing the most efficient sequence can save patients time and make smarter use of ED resources. Software can now reveal historic delays and show providers in real time each patient’s journey through the department and wait times. This allows providers to eliminate recurring bottlenecks and call for staff or immediately reroute patient traffic to improve efficiency.
ED To Inpatient-Bed Transfer
Helping providers forecast the likelihood that a patient will need to be admitted and present an immediate estimate of which unit or units can accommodate them is another excellent example of how predictive analytics can be game-changing. With this information, the hospitalist and ED physician can quickly agree on a likely onboarding flow, which can be made visible to everyone across the onboarding chain. This data-driven approach also helps providers prioritize which beds should be cleaned first, which units should accelerate discharge, and which patients should be moved to a discharge lounge. Using a centralized, data-driven patient logistics system, Sharp HealthCare in San Diego reduced its admit order-to-occupy time by more three hours.
Accelerated Discharge Planning
Getting patients admitted and scheduled for treatment is only half the battle. To optimize discharge planning, case managers and social workers need to be able to foresee and prevent discharge delays. Electronic health records or other internal systems often gather data on “avoidable discharge delays” — patients who in the last month, quarter or year were delayed because of insurance verification problems or lack of transportation, destination, or post-discharge care. This data is a gold mine for providers; with the proper analytics tools, a provider can predict with fairly high accuracy who among its hundreds of patients is most likely to run into trouble during discharge — all within an hour of a patient arriving and completing their paperwork. These tools help case managers and social workers create a shortlist of high-priority patients whose discharge planning they can start as soon as the patient is admitted.
Making excellent operational decisions consistently, hundreds of times per day, demands sophisticated data science. Used correctly, analytics tools can lower healthcare costs, reduce wait times, increase patient access, and unlock capacity with the infrastructure that’s already in place.
About The Author
Sanjeev Agrawal is president and chief marketing officer of LeanTaaS, a Silicon Valley-based innovator of predictive analytics solutions to healthcare’s biggest operational challenges. He works closely with dozens of leading healthcare institutions including Stanford Health Care, UCHealth, New York-Presbyterian, Cleveland Clinic, MD Anderson and more. Sanjeev was Google’s first head of product marketing. Since then, he has had leadership roles at three successful startups: CEO of Aloqa, a mobile push platform (acquired by Motorola); VP product and marketing at Tellme Networks (acquired by Microsoft); and as the founding CEO of Collegefeed (acquired by AfterCollege). Sanjeev graduated Phi Beta Kappa with an EECS degree from MIT and along the way spent time at McKinsey & Co. and Cisco Systems. He is an avid squash player and has been named by Becker's Hospital Review as one of the top entrepreneurs innovating in healthcare. For more information on LeanTaaS, please visit https://leantaas.com/ and follow the company on Twitter @LeanTaaS, Facebook at www.facebook.com/LeanTaaS and LinkedIn at www.linkedin.com/company/leantaas