How The 360-Degree Patient View Drives Data-Driven Care Decisions
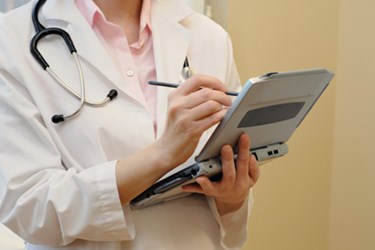
By Eric Sullivan, Senior Vice President, Product Innovation & Data Management, Inovalon
It’s no secret. The way most of us make important healthcare decisions is fundamentally flawed. Rather than conducting the type of rigorous research befitting a potentially life-and-death decision, which would allow us to make the most informed decision, we ask people close to us for advice or recommendations.
Why do we do this? Because when we start to peel the onion, we quickly experience a data avalanche of disparate and disconnected data points, a wide array of information that may or may not be relevant to our individual situation, but nothing resembling “the truth,” and often nothing valuable enough to help us make a decision. And we get overwhelmed. This isn’t unique to patient care decisions.
Similarly, clinicians across the care continuum need access to timely, relevant insights in order to see a true 360-degree view of the patient, ensuring that care decisions are based upon the most pertinent, complete and timely patient data. Bringing together disparate data sources enables highly granular, patient-level insights for the successful transition from volume to value.
Across the U.S., healthcare organizations of all types are impacted by evolving regulations and policies at both the state and federal level. To remain compliant, they must have an infrastructure in place that offers the flexibility to adapt quickly alongside new standards and requirements. Platforms that include advanced technologies, such as Natural Language Processing (NLP), Machine Learning (ML) and other forms of Artificial Intelligence (AI), empower clinicians and organizations to meet the unique and varying requirements of regulatory bodies and ultimately improve patient care, outcomes and wellness.
Leveraging Interoperability With Natural Language Processing
With the application of NLP, medical groups that are “taking risk” from CMS and other payers or are participating in payment programs such as the Merit-based Incentive Payment System (MIPS) can benefit by greatly reducing the need to exhaust often scarce and costly resources to manually read and analyze thousands of patient medical records for data submission audit requirements by CMS and other regulatory authorities.
Applications of Natural Language Processing — powered by Machine Learning — can now be used to evaluate the relevant clinical information found within medical records with a greater efficiency and completeness over the traditional approach of human clinical review of often lengthy medical records, delivering bottom-line savings in the process.
Furthermore, many platforms enable medical groups to parse structured and unstructured data found within a continuity of care document (CCD), which further improves their efficiency and the completeness of the analytically driven patient profile upon which the clinical teams rely.
Accelerating The Value Of Quality Healthcare Data
Today's Machine Learning platforms expand upon the classic regression techniques that are found in traditional predictive analytic engines to derive a more dynamic, patient-specific understanding of health care systems and, most importantly, individuals. For data scientists, however, the “Holy Grail” is doing all of this in real-time in a broad-spectrum manner leveraging disparate, big data. The ability for a computer system to analyze thousands of disparate and evolving data points (i.e., Big Data’s high volume, variety and velocity trifecta) to create patient-level predictions driving more precise treatments is a game-changer for many clinical conditions.
Empowering Clinicians With On-Demand, Real-Time Data-Driven Insights
As the dependency on data for quality and measure performance insights, reporting, and point-of-care services expands, clinicians are challenged with extracting, analyzing and managing enormous amounts of information about their patients.
Upon bringing together massive scales of disparate data sources into one common location, real-time calculations of a comprehensive patient profile can provide patient-specific analytics with actionable insights. Ordered on demand by clinicians at the point of care, this intelligence can identify and address gaps in quality, utilization, and medical history, supporting improvement in clinical and quality outcomes and economic performance across the healthcare ecosystem. While many organizations strive to do this in the workflow, most still rely on “outside” systems that aren’t quite integrated into how the medical group — from the medical assistant to the clinician to the referral coordinator when you check out — for support.
Conclusion
Ultimately, clinicians want to — and should — spend more time with patients. Relevant and focused data-driven insights can allow clinicians to address this need, but there remains a steep learning curve for some. Wouldn't it be nice if nearly every “data point” related to a patient was aggregated and analyzed to inform and thus improve the patient-specific quality of care delivered? As this happens, we will undoubtedly be far along in the transition to value-based care and a true 360-degree patient view, and Natural Language Processing and Machine Learning-related technologies will be a big reason for its progression.