How Cognitive Probability Graphs Can Save Lives
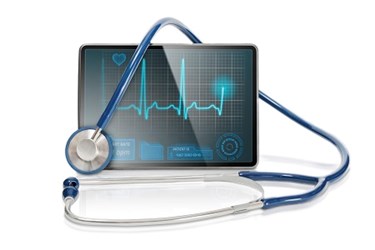
By Dr. Jans Aasman, CEO, Franz Inc.
Six months ago, my friend's young son — who was diagnosed with asthma — ate his first spoonful of peanut butter. Approximately 30 minutes later he was in the emergency room; the doctors barely saved his life.
Afterwards, the physician reviewed his medical chart and noted his peanut allergies were predictable because he also had asthma and dermatitis. My friend was understandably upset that this “prediction” occurred after his son's emergency room ordeal — especially since he was never tested for peanut allergies.
In the near future, it will be possible for patients throughout the healthcare industry to understand the probability of susceptibilities based on their genes, medical records, family history, and current medical condition. By combining artificial intelligence, semantic technologies, Big Data, graph databases, and dynamic visualizations — cognitive probability graphs can determine the likelihood of future medical events.
The ramifications of this technology could extend across the healthcare field to include pharmaceutical testing and development, life sciences, and virtually any other healthcare segment. Most importantly, they imply medical personnel would be able to identify relationships between conditions, prepare for them, and treat patients with a preventive efficacy that would transform the healthcare industry.
Artificial Intelligence
The power of cognitive probability graphs stems from the capability to combine the probability space — statistical patient data — with a knowledge base of comprehensive medical codes and a unified terminology system. Integrating these into a semantic graph enables a dynamic querying profundity that is otherwise not possible.
The cognitive probability graph process spawns from machine learning, one of the most accessible components of artificial intelligence. Once the relevant patient data is collected, machine learning algorithms calculate the co-occurrence and oddsratio’s between medical conditions or diagnostics. Co-occurrence is a fundamental concept in machine learning and can be simply understood as a number indicating how often certain objects occur together. In this case we look how often diagnostics are found together between patients. Oddsratio is a number that expresses how “unexpected” a co-occurrence is. A low oddsratio between two objects, in our case, between two diagnostics, means that the co-occurrence that we see might be expected given the overall frequency of each of the diagnostics in the entire population. A high oddsratio means that the co-occurrence that we see is much higher than might be expected given the distribution of the original diagnostics in the entire population.
Semantic Graph Databases (SGDB)
The integration of the probability space within the knowledge base — which begets enhanced querying capabilities — is directly attributable to the underlying SGDB in cognitive probability graphs. Semantic technologies convert odds ratios into standards based RDF triples, which are then used to determine additional conditions (or objects for whatever application the graph is used for) that are related to the initial one. The likelihood of additional conditions is quantified so that users know which ones are more imminent than others.
Social networking algorithms are deployed to provide the shortest path between any two diagnostics in a space of oddsratios, or probability space. Best of all, the unified terminology system enables users to see the various hierarchies of the conditions or procedures that are linked to specific events. These taxonomies yield even more insight into the relationship of medical conditions with one another, and facilitate a contextualization of co-occurrences that can greatly contribute to how medical issues are treated or prevented.
Visualization Mechanisms
Still, the most discernible boon of a cognitive probability graph is immediately obvious when combined with visualization capabilities, such as those provided by a platform called Gruff. When the aforementioned query process is simplified with visualizations, users instantly see the numeric values of co-occurrences and even more powerful - how they relate to each other. The graphic nature of these queries enables data discovery of different objects (medical conditions) that users would not be aware of otherwise — simply because without such visualizations, they would require a preconceived set of objects in mind prior to querying.
With them, however, users can view a hierarchy of medical conditions and visualize the plausibility of the strength of relationships, which events are most common, and the most probable sequence of events. The probability space provides the raw data, while the knowledge base illustrates different types of the same events to determine even more similarities.
Practical Possibilities
Cognitive probability graphs are remarkable not just because of the possibilities they engender, but also because of their practicality. The confluence of machine learning, semantics, visual querying, graph databases, and Big Data not only displays links between objects, but also quantifies the probability of their occurrence. These practicalities are invaluable for health services and can facilitate preventative measures that can transform systems from sick care to those of salubrious maintenance. Such transformative possibilities — and their pragmatic realities — exist across verticals, and can determine probabilities and preventative forms of customer churning, successful marketing measures, and other relevant events in additional verticals.
About The Author
Dr. Jans Aasman, Ph.d. is the CEO of Franz Inc. Dr. Aasman’s previous experience and educational background includes: KPN Research, the research lab of the major Dutch telecommunication company; tenured professor in Industrial Design at the Technical University of Delft; Carnegie Mellon University –visiting Scientist at the Computer Science Department of Prof. Dr. Allan Newell; researcher at the Traffic Research Center of the University of Groningen (The Netherlands); experimental and cognitive psychology at the University of Groningen, specializing in psychophysiology and cognitive psychology.