Healthcare Outcomes Measurement For Continuous Improvement
By Alex Trahey, Wellframe
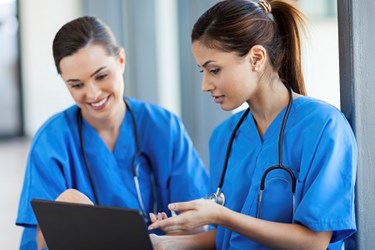
Measurement is an increasing priority in order to quantify every aspect of the business and demonstrate tangible value for health plans -- particularly in this digital age. This is especially true for health management programs, where evaluation practices have traditionally been unable to quantify impact.
Though it may appear overwhelming, it’s possible for organizations to harness the data collected from their health management efforts to more effectively quantify value. This piece will look at various measurement strategies that organizations can implement to demonstrate the merits of rigorous evaluation and continuous improvement—efforts that are imperative for achieving better outcomes and driving quality-based revenue.
Prioritize Outcomes To Define Success
At every level of health management, data is becoming more accessible than ever before—opening the door for data-driven decisions that can improve the service from bottom to top.
So, what will those improvements look like—and what measurements should you prioritize? As we know, many factors influence outcomes, from the number of patients enrolled in health management, to engagement rates over time, to quantifiable value created across dimensions such as patient experience, cost, and quality.
First, it’s important to define success relative to outcomes that matter. Then, choose the right metrics to back them up. In our experience, the granularity of measurement typically parallels the frequency and scale of the metrics in question. For example, measuring patient activity lends to daily or weekly review and improvements at the individual level, while evaluating operational workflows and efficiency metrics is suited to weekly and monthly evaluation and enhancements at the care team level. Considering overall program effectiveness, outcomes, and performance is appropriate for quarterly and annual analysis, and holds the potential for wide-ranging advancement at the program level.
Using Modern Statistical Methods
In order to fully understand the types of measurement discussed above, it’s important to recognize the methodology that goes into them—both the data sources and the analytical methods applied to that data. Limitations of traditional data practices stem from incorrect inferences of cause and effect, use of observational data, and inexperience in applying analytical methods. Many methods utilize single data sources that reduce the nuance of real patients or populations to insufficient numbers. However, programs can achieve more reliable and insightful results when they thoughtfully measure data and take a more rigorous approach to analytics methodology.
When you combine multiple analytical frameworks and sources, you can better understand any metric. By applying modern statistical and population health methods across multiple data sources, you can estimate impact on cost and utilization. Propensity score matching allows you to approximate randomization, finding similar groups of patients based on clinical and behavioral characteristics, and compare outcomes.
Leverage natural experiments to provide another approximate method of randomization that is applicable at the individual level as well as the care team and program levels. This setup provides a natural set of randomized groups for comparison, similar to having a control group and a “treatment” group. It allows us to discern cause and effect to uncover valuable insights about the treatment—insights that translate into tangible next steps.
By applying these comprehensive, rigorous measurement methods, organizations will be able to paint a holistic picture of member groups that more accurately captures their individual nuance. Moreover, this measurement methodology scales easily to quantify and understand a population, care team, or program as a whole.
Quantify To Continuously Improve
With novel data sources, sophisticated methods, and key metrics at every level of the health management program, health plans will be data-rich. However, data for the sake of data is fruitless; it must be grounded in learning and tied to action.
Measurement enables health management programs to continuously identify areas for improvement, implement changes, and measure the effect. The following examples are tangible ways data-driven improvements can take place from the individual patient level up to the executive board room. By scaling up processes that work and revising processes that don’t, programs can make meaningful progress toward end-to-end optimization.
Real-Time Interventions
A care manager noticed one patient’s falling medication adherence, and reached out to ask about the issue. The patient explained she didn’t take her pills when she traveled on the weekends. The care manager mailed a new pill box, and her patient’s medication adherence rebounded to normal.
Daily Improvements
Population reports indicated low comprehension of safe acetaminophen dosage. This finding combined with the risk of misunderstanding medications prompted a change in health education delivered directly after discharge to focus on safe dosing, resulting in an increase in patient-reported level of understanding.
Weekly Staffing Optimizations
Supervisors reduced the number of care managers focused solely on outreach for gaps in care when they noticed low patient satisfaction compared to a population in which care managers worked with patients more holistically, closed gaps more effectively, and saw higher satisfaction.
Monthly Outreach Adjustments
Claims and patient self-reports revealed falling attendance at PCP appointments. Care managers addressed this issue by switching to mobile channels to contact members before appointments and increase the frequency of reminders. Attendance rebounded to a higher rate than the baseline.
Quarterly Care Team Reassignments
With newly implemented technology, supervisors recognized tech-savvy staff early on and embedded them among less adept peers to share their tactics, bringing the whole group up to speed faster and with more camaraderie.
Yearly Reinvestment In Health Management
After showing thousands of dollars in cost savings per member, executives increased the budget for health management to support increased recruitment efforts and extend health management services to more members in order to double down on those results across a broader population.
Improving Outcomes In Health Management
With data to demonstrate effectiveness, impact, and value to the health plan, executives will pay attention. Health management leadership will be able to not only justify increased investment to grow the program even further, but also apply the same data models to effectively predict the return on additional funding.
Ultimately, rigorous measurement and continuous improvement enables health plans to both improve outcomes and quantify the impact of their efforts.
About The Author
Alex Trahey is a data scientist focused on helping healthcare organizations leverage their existing data to unlock opportunities for continuous improvement. He is the Director of Analytics at Wellframe, a patented health management solution that supports patients’ health beyond the four walls of care delivery. Prior to his work at Wellframe, Alex conducted health outcomes research at Analysis Group, where he co-authored numerous articles on topics including rheumatoid arthritis, sickle cell disease, and herpes zoster.