Healthcare Data Warehousing: Managing Many Moving Parts
By Mahesh Dedhia, Senior Manager, CitiusTech
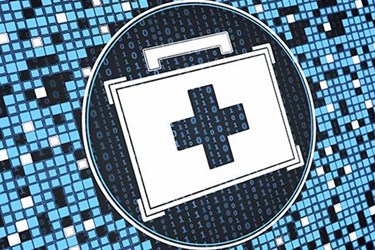
With the healthcare industry seeing a massive shift towards consumerism and advanced analytics, getting a single, comprehensive view of healthcare data is becoming imperative. This has made data warehousing more valuable than ever, which is why many leading healthcare organizations are beginning to implement enterprise data warehouses.
At the same time, most organizations also realize that data warehousing projects are infamous for scope creeps and missing deadlines due to various reasons such as performance gaps, data integration challenges, and discovering new scenarios and use cases mid-way. For healthcare organizations striving to constantly improve patient outcomes and reduce costs, it has become important to make effective use of the data gathered over the years to increase operational efficiency, optimize infrastructure investments and improve customer experience. Data warehousing professionals often need to sift through terabytes of data from legacy applications and decipher data elements for advanced analytics.
To implement an enterprise data warehouse, it is important to understand these challenges early on so projects can be realistically planned to deliver against well-defined goals. Here are some of the key guiding principles that data warehousing professionals need to consider while planning for and building healthcare data warehouses.
Part 1 — Address Source System Disparity. Data warehouse implementation teams need a deep understanding of source systems such as electronic health records (EHRs), Health Information Management Systems (HIMS) and claims processing systems. Teams can gather this information from business subject matter experts (SMEs), source system documentation and data dictionaries.
In the absence of documentation, SMEs become crucial assets who can ensure a successful data warehouse implementation. In a provider organization, SMEs are often associated with the IT or the configuration team, not the core warehouse implementation team. Management should understand its dependency on the SMEs and commit necessary resources to the implementation team.
Some payer and provider organizations that have built separate data warehouses end up managing data from different sets of source systems. Since payer and provider data warehouses vary drastically, integrating these warehouses requires reconciliation of key entities such as patients, health plan members and encounters. This can be achieved by a collaborative effort of SMEs from multiple source systems and warehouses.
Part 2 — Create a Sustainable Architectural Construct. Immediately after selecting the right technology and hardware to defining the data model, careful and deliberate effort is required to make the proper decisions around data warehouse architecture and Extract-Transform-Load design (ETL).
While selecting a specific deployment model, either on-premise hardware infrastructure, Infrastructure as a service (IaaS) or Analytics as a service (AaaS), organizations need to be acutely aware of various ecosystem needs, such as regulatory compliance, performance and availability benchmarks, and security needs.
Part 3 — Select a Vendor. The choice of vendor matters greatly in implementing a successful data warehouse project. Depending on specific organizational and IT goals, organizations may choose to procure best-in-class tools and services from specialized vendors or work with a single vendor that has a strong understanding of healthcare workflows and provides the necessary tools and services.
Often, these overarching considerations may fall outside the scope of the data warehousing project. High-level architecture and low-level design decisions are largely constrained by these boundaries and require effective decision-making at various levels of executive management, including the CTO, CIO, CMIO, Head of Quality and CFO.
Part 4 — Align Team Structure to Execution Models. The choice of project execution model depends mainly on the organization's comfort level and maturity with the model. While systems development life cycle (SDLC) is still a widely-used model, Agile works better when team members are dedicated to the project.
The execution model also influences the team structure, in terms of roles, proportion of skills required and time commitment of team members. In addition to a dedicated team composed of developers, business or data analysts and quality assurance engineers, it’s important to have a few key roles such as architect, DBA, source system administrators and source system SMEs on the team during project execution.
Part 5 — Focus on Security and Usability. Since HIPAA and HITECH laws have defined strict policies around guarding protected health information (PHI), organizations need to consider a few additional factors:
- Cloud versus on-premise infrastructure;
- Use within the organization's intranet or virtual private network (VPN); and
- Exposure of interfaces over the internet, while defining security protocols.
Identifying the right set of business users and creating a structured approach to user acceptance is important to avoid project delays. Organizations would benefit from uncovering potential issues during user acceptance testing (UAT) and increase adoption within the user group if they incorporate a mix of clinical users who are familiar with source systems and workflows, and healthcare analysts who are responsible for deriving insights.
Part 6 — Manage Change Effectively. In today’s evolving market, data warehouses cannot keep up with the slew of change requests. It takes even greater time to decide whether the change request can be postponed or accommodated within the project scope and timeline.
At times, delaying a change request may result in missing out on a data entity, letting incorrectly-mapped data flow into the warehouse, or even pushing certain transformations downstream (at the time of fetching data from the warehouse). In such cases, a product owner can help make appropriate decisions. By evaluating the business impact and being creative about managing the change, the impact on the scope and the schedule can be minimized.
Recommendations for Provider and Payer Organizations
Managing the many moving parts involved in a healthcare data warehousing project can be a daunting task. Apart from the guidelines mentioned above there are several other factors that come into play, such as daily data volumes and load frequencies, master data management (MDM) implementation and setting up the data model for self-service business intelligence (BI). Without the required healthcare and technical expertise and the right team, it can be an expensive, time-consuming affair, and the outcome may not be optimal.
At the same time, with constantly evolving analytics and data warehousing technology, organizations will find newer ways to leverage data for strategic decision making. Data warehouses, despite the challenges involved, will continue to be a powerful, strategic tool for healthcare organizations in the years to come.
About The Author
Mahesh Dedhia is a Senior Manager for CitiusTech.