Data's Credibility Problem: It's About The Quality, Not The Data
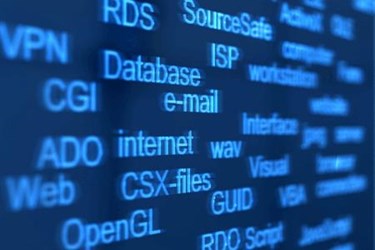
By Syed Haider, senior architect, X by 2
In the first part of this article, we discussed the ways in which health insurers should begin to position themselves for the transformation to a data world where the quality of the data — and thus its believability — holds primacy above anything else.
In today’s ultra-competitive health insurance landscape, the quality of one’s data is akin to an information-based competitive advantage. That’s because it’s not just the data itself that provides actionable and profitable insights, it’s also all the other ancillary costs embedded in poor data quality in an insurer.
And what are those costs? It’s a long list, but a good place to start is the cost of resource redundancy in an insurer when multiple departments attempt to produce or reproduce the same reports from differing data sets. This is something that happens on a daily basis in almost every health insurer.
A more direct cost to any insurer dealing with poor data quality is the cost expended to correct and reconcile inaccuracies in the reports generated from poor quality data before those reports see the light of day. This can be a significant resource burn for any insurer. One other cost that’s worth mentioning is an elusive but potentially impactful one — the cost of lost opportunities for insurers who might never even know an opportunity for marketplace advantage exists at a point in time because their poor data never tells them that.
All of these reasons and more argue for insurers to take an enterprise approach toward a transformational data quality initiative. That approach begins with acknowledging the need and continues with the sort of broad executive support that gives the initiative the proper priority in the insurer.
The executive support should go a long way toward mobilizing the resources of the insurer behind the data quality initiative. It should also position the initiative where it rightly belongs in the insurer — at or near the top of the corporate priority list.
And why should a data quality initiative leapfrog over what are inevitably the multitude of other business technology priorities at any insurer? Because at its core, data quality is something that is difficult to grasp, but most people recognize it when they encounter it — that’s believability. It’s too often the case that data believability is questioned in insurers, often on a daily basis, and that leads to credibility issues for IT and anybody else involved in the production of the poor data. It can also lead to embarrassing missteps in the market, or worse yet, missteps directly with providers or customers.
A soundly implemented data quality and governance initiative makes the data believable, and that’s worth a lot to any health insurer.
The devil is always in the details of course, and the next step for any insurer is creating and organizing a data quality program than can be realistically be achieved, and be successful in the eyes of any and all stakeholders.
A good place to start is by identifying the best opportunities for data quality success in the company. Where can the insurer get some quick but important wins in the data quality march as a way to build and increase momentum for the initiative? This is important on several levels.
First, it allows the insurer to get their feet wet with the tools, techniques and processes required for a strong data quality initiative. Many insurers start by creating a useful executive dashboard as a way to socialize the potential for data quality, and to help company executives visualize what a sound data quality approach can bring to the company.
Second, early success opportunities are a good way to begin to create the metrics around data quality that will ultimately turn into a solid governance approach that ensures that once established, that data quality platform never backtracks.
And third, early successes will go a long way toward establishing what success actually looks like. That helps to get people used to the iterative process that data quality entails, and helps to build confidence and credibility in the actual data itself — back to the believability issue.
All of this is followed by the nitty-gritty of data quality programs: business rules, process, technology, and governance. This is especially true when it’s the first time through the data quality process for an insurer. That’s the best time to think ahead and put a repeatable and sustainable data quality resolution process in place.
It is a truism that as soon as the data quality program is implemented in its initial phases, there will be data credibility issues to address. That’s why it’s a good idea to map out the reactions and resolution processes up front, starting with the question of: what is the nature of the data quality problem? That’s important because the insurer wants to always be sure that they’re matching the appropriate level of response to any particular data quality problem.
Is the problem a historical one that will require a massive investment in resources to correct? If that’s the case, should the data just be recreated as new? Is the nature of the data problem an ongoing or transactional one? If that’s the case, a more modest resolution approach might be taken. However, if the nature of the data quality problem is that it’s creating a lack of clarity or believability in the data, then that warrants a substantial and rapid response. A best practice approach is to create data quality “triggers” as part of an overall data governance approach, mapping and documenting appropriate responses to each data quality “trigger” event.
Finally, it’s imperative to mind the old management adage that one can’t manage what one can’t measure. That’s especially true in data quality programs. A measuring and reporting structure must be created to map the progress of the insurer’s data quality program across time. The measurement and reporting structure should be multi-dimensional, including such elements as measuring for qualitative improvement, measuring for signs of data quality erosion, measuring the insurer’s overall data quality learning process to determine effectiveness, and any measurement and reporting approach should be overlaid on top of any new project or initiative in the insurer. That helps to ensure the institutionalization of the data quality program so that it becomes part of the insurer’s DNA, and can be passed along to subsequent employee generations at the insurer.
It’s not a small matter to implement a sound and sustainable data quality program in the healthcare industry. The industry is strewn with insurer’s who have gone down this path only to see their efforts thwarted by the state of their current data, the technology chosen, the shifting budget and resource priorities, and even the ingrained culture of the insurer. All of that doesn’t mean it’s not worth doing because in the end it emphatically is.
The future of the health insurer market will be composed of the haves and the have-nots — those who can effectively wield actionable data will be the long-term winners in the marketplace.
About The Author
Syed Haider is a senior architect for X by 2, a technology consultancy focused on the practice of architecture for the insurance and healthcare industries based in Metro Detroit.