Data Analytics – The Hidden Gem In Helping To Combat The Opioid Epidemic
By Richard K. Grape, LexisNexis Risk Solutions
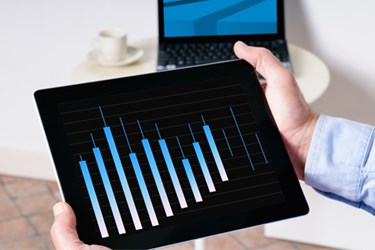
This is the first of a two-part series that discusses the role of data analytics in helping to fight the opioid epidemic.
The opioid epidemic continues to threaten the public’s health, wellbeing, and ultimately, its wallet. With opioid-related overdoses resulting in 116 deaths per day, the epidemic has become a public health emergency. The total “economic burden” of prescription opioid misuse alone in the United States is estimated around $78.5 billion a year. The costs of this problem extend beyond just healthcare, including those tied to lost productivity, addiction treatment and criminal justice involvement, as well as the many social costs that are challenging to quantify. The epidemics effects are being felt by commercial healthcare, pharmacies, Government agencies and programs, and every industry who employs its victims.
There is no one path to avert this crisis, but industry stakeholders have a unique opportunity to come together and leverage new technology and tools to identify, mitigate and manage current and future situations of opioid abuse. So how can Big Data and analytics help healthcare reduce health risks and stop fraud, waste and abuse? It starts by identifying and evaluating the entities who contribute to potential risk, or who are at risk, within the various stages of the healthcare ecosystem.
Using Data And Analytics To Surface High-Risk Entities In Order To Reduce Their At-Risk Status
Patients or members are the most at-risk group. These include individuals who are opioid naïve, e.g. those that are new to taking this prescription type, those who are taking prescriptions that may have cumulative effects, individuals who may be intentionally abusing prescriptions or partaking in recreational drug use, as well as those potentially engaged in resale of these drugs on the black market. Technology can help aggregate prescription information from multiple sources, but it must be coupled with robust identity resolution in order to effectively identify individuals at-risk.
Providers or prescribers are the second source of potential risk. Some of this group’s risks include seemingly innocuous behaviors that merely require reeducation. For instance, prescriptions may be inadvertently written to a number of high-risk patients or even to patient family members. However, some provider-driven risks may be intentional such as writing prescriptions to their friends and family members or writing prescriptions in excessive quantities for certain drug types. Data aggregation and analytics can help surface these data points and flag situations of provider risk.
Pharmacies represent the third group of potential risk. Similar to the provider group, pharmacy risks may be inadvertent or intentional. Inadvertent risks include targeting by patients who seek to fill manufactured or fake scripts, as well as targeting due to less robust patient/provider screening prior to script filling, resulting in easy filling. Data and analytics can help surface these instances by comparing local pharmacy fill rates to evaluate how one pharmacy differs from its peers. Analytics also can surface situations where high volumes of patients who seek prescriptions at a single pharmacy all originate from a single doctor, or even where prescriptions are not associated with a corresponding medical event, such as a doctor or hospital visit.
Patients, providers, and pharmacies represent most of the high-risk and at-risk entities of focus. However, there is one other risky group that can only be exposed through technology, data, and analytics…
Social Groups are at the heart of the opioid crisis and the largest source of potential risk. Social groups represent the clusters or networks of individuals who work together to drive widespread drug diversion. It is only by evaluating relationships outside of the normal scope of healthcare data that these networks of entities who act with a singular purpose can be identified. Social groups require outside data sources to be surfaced as they look at friends, family members, business colleagues and close associates.
Why should the industry focus on Social Groups? According to the Centers for Disease Control and Prevention (CDC), drug diversion, the transference of legally controlled and prescribed substances from one individual to another, is a primary avenue for opioid abuse. Socialization can only be identified through entity resolution, taking those tied to healthcare interactions, layering their footprint and network as identified through public record data assets, and surfacing entities and clusters of potential abuse and/or mal-intent.
4 Critical Measures Only Data Can Reveal
"Pill mills” is a term used by investigators to describe doctors, prescribers, clinics or pharmacies that are prescribing or dispensing a powerful drug either inappropriately or for non-medical reasons.
Identifying pill mills is hard, but critical for your investigations. This can be identified by:
- Surfacing prescribers who are treating high percentages of patients receiving high-risk drugs
- Surfacing pharmacies who are filling excessive scripts for patients receiving high-risk drugs
- (Most importantly) Surfacing where prescribers or pharmacies may be providing drugs to a large social network of patients who are organized and working together to obtain them in large quantities
“Frequent Fliers” and “Doctor Shoppers” are patients who go to one or multiple providers in quick succession with the goal of obtaining prescriptions for high-risk drugs.
These individuals typically seek illicit drugs for:
- Abusive drug habits
- Drug diversion for resale/abuse
- Drug diversion to relatives/associates
Frequent Fliers and Doctor Shoppers are particularly difficult to identify due to the short time frames in which they frequent multiple prescribers, through identity misrepresentation, or by paying cash.
“Socialization” takes many forms, but includes relative and associate relationships, participation in one another’s social network, providers who are part of their patients’ social network, and patients who are part of other patients’ social networks. Socialization targets include high-risk patient, provider, and pharmacy networks and by mapping relationships, healthcare can easily identify the social groups to which these high-risk entities belong. Each member’s risk can draw greater attention to the risk of the whole, and actors who might otherwise go unnoticed suddenly become obvious participants in an organized scheme.
“Unsafe MEDs” calculations can provide transparency into other unknown drugs that a patient is receiving which can play an important role in averting opioid overutilization. Patients who receive one or more high-risk drugs that result in unsafe MED totals indicate potential situations of diversion, abuse, or health risk. Tracking daily patient and social group MED totals can help identify individuals/networks who are at high-risk of perpetrating drug diversion schemes. Identifying these groups can ultimately minimize the potential for prescription drug abuse/misuse and reduce the number of unintentional overdoses associated with pain medications.
In part II, we will look at how bringing together different data assets can:
- empower unprecedented decision making and
- help health stakeholders to Prevent, Detect and Collaborate to address known or emerging risks as they engage with the different entities throughout the healthcare industry
About The Author
Richard K. Grape, Jr. is the director of market planning for LexisNexis, overseeing efforts in the areas of fraud, waste and abuse for the Health Care business. In this role, Mr. Grape is a strategist that directs the overall market, product, vision and sales strategies for the company.