Can Whole Person Analytics Improve Health Equity?
By Josh Morgan, PsyD, SAS
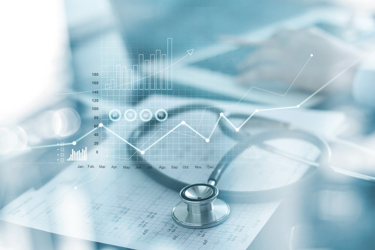
Identifying and dealing with health equity is reliant upon reacting to retrospective data and insights. Could we use modern technology to respond more proactively to help vulnerable populations?
As the world battles a global pandemic, COVID-19 has strongly demonstrated the fundamental need for true holistic data platforms. We have seen the life-impacting consequences of not bringing data together for a whole person view of the community—identifying the needs and the impact of interventions. Health inequities are exacerbated as a result of this failure, especially in vulnerable populations.
To address this, employing flexible data platforms that enable whole person risk stratification can empower more nimble responses to improve human outcomes, including equity. In this model, risk stratification allows data-driven identification of people at higher risk and need. Central to this though, is a data platform that respects both privacy and ethics, especially when attempting to improve health equity. We all know the serious consequences of unethical AI and breaching privacy. This is particularly egregious when trying to serve more vulnerable populations.
When analyzing the health impacts – and health inequities – of COVID-19, population-level insights are key. While we need PHI and PII to perform the analytics properly, they can be anonymized and not surfaced to end users individually. This approach provides needed insights while also protecting individuals’ privacy. Even when identifying individuals in need to drive prevention work, basic information like names and contact information could be provided to care management teams to facilitate interventions. In this way, PHI could be protected while still helping drive the risk stratification algorithm and identifying those in need.
Likewise, technology can raise awareness of gaps and needs, making human outreach more efficient in screenings. The human screenings and relationships are still important and empowered by more holistic information, we can build more compassion and empathy, driving a more ethical and equitable system. Privacy alone doesn’t guarantee ethical approaches, though. There are two ways whole person analytics can improve ethics:
1. Bring Holistic Data Together
There are many unique needs and systemic barriers for particular individuals and populations. One of the many causes of health inequities is not paying attention to contextual information, including what is often called social determinants of health, such as housing, education, and economic status. The integration of data allows us to see a more complete, accurate picture of both need and impacts.
By not paying attention to this information and using it to identify needs as well as outcomes, we are missing critical components of overall health and wellness and contributing to inequities. Instead, by looking at the “whole person” we can build more compassion and empathy, which can empower more equity.
2. Reduce Algorithm Bias
Algorithm bias is a significant problem and barrier to using advanced analytics to improve health equity. By using more whole person data as well as transparent algorithm development, we can more easily identify and control for biased variables. Since biased analytics and AI comes from biased data, which in turn comes from societal assumptions about people and how they behave, continuing to look at the same limited data will only reinforce those narrow views. When we can see the holistic impacts of our analytics, we can more quickly and accurately see where problems in risk stratification and other analyses may be occurring. We can surface assumptions we made about people and how they interact with the health system, likely identifying legitimate barriers to care. Further, with more complete data, we have the opportunity to improve our approaches and reduce bias in our algorithms and more importantly, improve our broader health systems.
As an example, in some of my prior work, our outreach and engagement program targeted people who were “resistant and non-compliant to treatment”—we essentially assumed certain negative things about people not activated into care. That pejorative assumption placed all the onus on the consumer receiving care, assuming they were the problem. However, as we began to explore the data even basic billing information helped challenge our negative assumptions.
Using that data along with observations from the care teams, we discovered people were trying to get care and it was actually our health system that was causing the majority of the engagement problems than the individual consumers themselves. We did not have a system that could be accessed easily and appropriately by the people in most need. We structured things for our own sake and business operations, not for the needs of the vulnerable.
An important component of analytics, AI, and data generally is the opportunity for self-reflection. The best insights from data don’t come from telling us what’s wrong with other people. As a psychologist, I can tell you we can’t change others. Rather, helping us see our blind spots and opportunities for improvement allows us to change ourselves, both personally as well as systemically. And that has a direct impact on lives. Let’s join together to gain more whole-person insights to help us all move forward to a more equitable and healthy society.
About The Author
Josh Morgan, PsyD, is National Director of Behavioral Health and Whole Person Care at SAS, where he helps health and human service agencies use data and analytics to support person-centered approaches for better health outcomes. He is a #data4good evangelist and advocate for improving benefits, access to care, and more holistic services. Find him on Twitter, @DrJosh.