Artificial Doctors In A Human Era
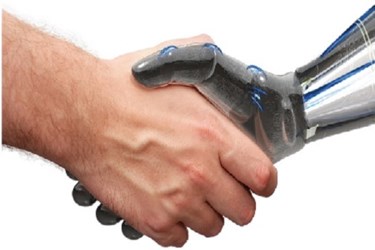
The term Artificial Intelligence (AI) is overused today. Unfortunately, this often leads to a misunderstanding of what AI is. Artificial intelligence is an umbrella term and covers many areas, including robotics, machine learning (ML), natural language processing, knowledge representation and computer vision. For the purposes of this article it will suffice to view AI in a simplified form as a synonym of Machine Learning with one stipulation – AI implies automatic decision making while Machine Learning only provides insights for a human observer to facilitate the decision-making process.
In 2014 the population of the Earth exceeded six billion. All those people need medical help and care with varying degrees of urgency. Today, it is well understood that preventing diseases is more cost effective than treating them. According to the 2017 report of the Milken Institute, “More than 109 million Americans report having at least one of the seven diseases...the total impact of these diseases on the economy is $1.3 trillion annually.” This number leaves no doubt that we must search for new ways of reducing healthcare expenditure. Accordingly, we must utilize the power of AI to solve many contemporary healthcare problems. An AI-based approach becomes even more attractive if we recall another consequence of the growing population – the rapid growth of the amount of medical information. The number of MRI scans, for instance, has tripled from 1997 to 2006. The number of lab tests increased similarly. Clinicians are inundated with medical data. It is scarcely possible for them to process such a plethora of information at a reasonable pace.
The situation is exacerbated by the shortage of doctors. According to the recent report prepared by the Association of American Medical Colleges, the shortfall will range from 34,600 to 88,000 physicians in 2025.
To date, the efficacy of old methods has been weakened, while the overall situation has become more complicated. At the same time, we have all heard about the rise and fall of AI in various areas like finance, legal, marketing, games and, ultimately, in medicine. Is AI capable of helping healthcare? This article covers the diagnostic capabilities of modern AI.
We intuitively believe that the chances of a doctor making an erroneous diagnosis and proposing an ineffective or harmful treatment plan are low. At least in our case. Unfortunately, the statistics about the accuracy of human doctors is disappointing.
Figure 1 Most common causes of death in the United States, 2013.
(Makary & Daniel, Medical error — the third leading cause of death in the US , BMJ, 2016)
According to the 2016 research, the third leading cause of death in the US is medical error, directly following heart diseases and cancer (See Figure 1).
Human doctors are prone to error. In some cases, errors occur due to distractions, in others they stem from the difficulty of obtaining information such as the patient’s medical history. Whatever the reason, an artificial assistant could diminish the influence of these factors.
An expert system is a general term that does not belong exclusively to medicine. In a broad sense, an expert system is “a computer system that emulates the decision-making ability of a human expert.”
Today the medical expert systems are often considered as part of a broader class of medical computer systems – Clinical Decision Support Systems (CDSSs). CDSSs operations extend far beyond diagnosing. Their typical functions include alert, remind, find information, select treatment, report, prognosis estimation, and diagnose.
The primary purpose of expert systems is to use experts’ knowledge to make decisions about new cases. To approach the reasoning ability of humans an expert system must not only make decisions but also explain them, show the “reasoning path” that lead to the decision. Another necessary component is the knowledge base that is used as storage of experts’ knowledge representation.
The most famous medical expert system is Mycin It was designed to help diagnose blood infections caused by bacterimia. It demonstrated a level of accuracy 10.5 percent higher than that of human doctors: 65 percent of MYCIN’s antimicrobial therapy prescriptions were classified by experts as acceptable compared to 55.5 percent of prescriptions made by human doctors.
An example of a medical expert system (Health Navigator) is shown in Figure 2. A modern CDSS provides a high level of flexibility for integration. Using an Application Programming Interface (API), it is possible to integrate a CDSS with third-party applications, thereby extending its functionality This idea is exemplified by the integration of CDSS and IBM Watson speech in Health Navigator. CDSS often requires a textual input which is inconvenient, even if possible. The integration of CDSS can process text and extract medical information on patient’s symptoms and conditions. It is worth noting, that the need to input text into the CDSS creates discomfort for everyone involved and may well be one of the main reasons for the low popularity of diagnostic CDSS among physicians.
Figure 2 A web interface of the Healthcare Navigator’s system.
The mathematical concepts underlying the existing medical diagnostic expert systems vary widely: Bayesian networks, Decision theory approaches, and heuristic models (rule-based, frame-based, case-based).
Diagnostic CDSSs have been found to improve the diagnostic performance of clinicians. Although the effect is not negligible, only a few studies demonstrated the benefits of diagnostic CDSS. Two explanations were proposed: one is that clinicians “are better able to rule out alternative diagnosis than CDSS” owing to their experience; another one is that diagnostic CDSSs require a substantial input of patient’s data, a possible reason why CDSSs are not used. In view of these conclusions, the idea to use voice to load the patient’s data into CDSS, as done with Health Navigator’s CDSS, is an attractive one.
The process of CDSSs adoption by clinics is slow. The healthcare system has a colossal inertia, while convoluted regulations create additional barriers. The desktop systems have failed to be quickly adopted by hospitals. Development of Web and mobile technologies facilitate the proliferation of the light weighted form of expert systems – symptom checkers (SC). The motivation for creating SCs was to replace the Web search engines, e.g., Google, as a source of self-diagnosis. The advice to seek emergency care was proposed by a search engine only in 64 percent of answers for emergency and urgent cases. Today, with a single mouse click, one can get a number of SCs – 143 symptoms checkers were identified through a Web search in a 2015 study. The role of SCs is two-fold: to improve the accuracy of self-diagnosis, and to improve the accuracy of practitioners by assisting with triage.
Symptom checkers tackle a complex problem, imposing high demands on their accuracy. The accuracy of 23 SCs was evaluated in a series of recent research from 2015 to 2016. Physicians demonstrated higher accuracy - 84.3 percent of participants gave the correct diagnosis among the top 3 most probable diagnoses - than the symptom checkers with 51.2 percent. Nonetheless, SCs can facilitate self-diagnosis in acute and urgent cases. Thus, the Health Navigator’s triage support engine can estimate the level of acuity and advice the user to call the emergency line.
Although less accurate than human doctors, SCs impact the probability of appropriate decision making by assisting triage. Thus, SCs provided an appropriate triage advice in 57 percent of patient evaluations as the study shows. Efficacy in advising triage is higher for emergency care patients.
While SCs do not provide an adequate substitute for a human diagnostician, they are an excellent alternative to Web search. Most of them prompt the user to find appropriate medical care and avoid self-care, thus, reducing the risk of complications in urgent cases. Moreover, in assisting clinical triage they positively impact physicians’ performance and accuracy.
AI incarnated in “artificial physicians” is in its adolescence and is not able to replace the human physicians yet. If circumstances are novel and the knowledge on new situations is lacking, AI is much weaker than the reasoning abilities of a human. It does not mean that AI is useless in general practice. We discussed the above cases when AI’s help is indispensable. The most prominent is an augmentation of physician’s skill in diagnosis reasoning and avoidance of the self-diagnosis in search engines.
Unfortunately, some obstacles hinder a broad adoption of AI in medicine. They have been precisely summarized by Edward Shortliffe as of “political, fiscal and cultural nature rather than technical.” Therefore, the challenge of approaching an advent of artificial diagnosticians is multi-faceted. History teaches us that the accuracy of AI is a necessary but not a sufficient condition for adoption. To succeed, the product must possess a nifty interface, must be accurate to win the confidence of its users and has to reason the same way we do.
Medical expert systems, so to say, “artificial physicians”, came a long way since their dawn – from a bloom of belief in AI in 60s, through the AI winters in 70s, 80s and 90s to today’s AI spring. We are waiting for AI summer to come.
About The Author
Anton Dolgikh leads AI and ML projects in the Healthcare and Life Sciences Practice at DataArt where he also conducts educational seminars on solving business problems with ML methods.
Anton’s background is in academia. Prior to joining DataArt he worked in the Department of Complex Systems at the Université libre de Bruxelles, one of the most prominent Belgian private research universities. Prior to that, Anton taught physics and mathematics at the Voronezh State University, where he also led scientific research and has published 11 articles on condensed matter physics, quantum mechanics, and statistical physics.
Anton holds a BS and MS degrees in Theoretical Physics, and a PhD in Condensed Matter Physics from the Voronezh State University in Russia.