AI Is Already Changing Healthcare, But Not In The Ways You Might Think
By Vinay Seth Mohta and Sailu Challapalli, Manifold
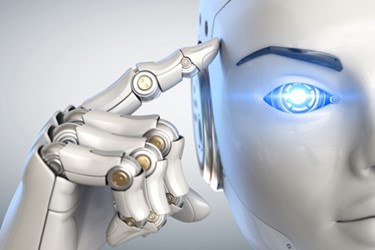
Artificial intelligence has enormous potential for disruption in many fields, particularly healthcare. Indeed, given the industry's complexity, scale, and runaway costs, AI presents an especially enticing opportunity. What healthcare leaders must understand, however, is that the technology itself isn’t a magic bullet. AI and machine learning cannot solve all of an organization's problems overnight.
As with other innovative technologies in healthcare, the key to capturing value from AI is having a clearly defined need and integrating the solution into existing workflows. That doesn't mean that AI will replace the human work that healthcare demands; rather, AI will be able to augment clinical and administrative staff's ability to engage and treat patients. For example, a deep learning algorithm can be used to identify certain factors that indicate a higher risk for non-compliant behavior from a patient.
What the technology can’t do (yet) is determine the best method of engaging the patient to ensure compliance and then actually work with the patient to change behavior. It’s up to care providers to integrate the findings from such algorithms at the point of service (i.e., when interacting with a patient). AI will be of the most use in situations like these, where the technology unlocks the value of a provider's insight and experience.
Popular AI Misconceptions
Healthcare leaders are sometimes tempted to take an “if you build it, they will come” approach to data and AI. They construct a massive data depository (sometimes called a “data lake”), which aggregates different types of data across the organization. They then share the data lake's capabilities with other business and operational leaders to identify potential business needs that can be solved using the data now available. It's quite the operation.
This approach might work for larger organizations that have the flexibility, resources, and time to invest in this often multi-year endeavor. For smaller organizations, however, this well-intentioned undertaking can be an example of putting the cart before the horse. An alternative approach is to start with a set of near-term business priorities that are specific and can be readily solved using AI and machine learning. Only once these areas have been identified and prioritized is it time to start aggregating the data, focusing specifically on the data elements relevant to the prioritized problems. Once these initial solutions have been successfully built with the help of AI, leaders can go about addressing other challenges. The best part? With each incremental win, leaders gather more experience and more buy-in from key stakeholders.
To help leaders get a head start, we’ve identified three use cases where AI could be used to achieve such incremental wins. All three of these examples can occur relatively early in an organization’s AI journey, as most of the data is already available. That means healthcare organizations can start moving on these wins right away.
Win #1: Routing To Appropriate Service Providers
Given that health systems are becoming increasingly large, complex structures with multiple access points and specialized providers, connecting the right patients with the right resources can be difficult. But by leveraging data from a patient's preferences and information about the providers within the organization, AI can match patients with the most appropriate care team.
Tools like chatbots can be incredibly valuable in integrating AI into operational workflows by automating standard portions of patient interactions. For example, chatbots can triage patients to the right service (e.g., telehealth, urgent care, a primary care office, or a specialist), schedule appointments, and streamline the intake process. With a recorded or transcribed interaction between patients and intake staff (or an intake chatbot), an organization can leverage natural language processing and other machine learning tools to automatically create a decision tree based on the initial automated interaction.
If automating patient interactions sounds insensitive, know that the goal of automation is to process — and help — as many patients and caregivers as possible in an overloaded healthcare system. Companies such as Sensely and Buoy Healthcare are even using AI to make suggested diagnoses to patients based on the symptoms they describe or photograph. Machine learning tools are powerful, and they're getting more powerful all the time.
Win #2: Understanding Patient Journeys And Lifetime Value
Understanding a patient's journey through the complex health system and calculating a patient's lifetime value can seem daunting to healthcare providers. To do it comprehensively requires an ongoing process of aggregating data across CRM systems, patient portals, appointment scheduling tools, electronic medical records, and patient billing systems. But, more to the point, many providers might not trust a lifetime value calculation in an industry where costs and revenue are difficult to predict — how, they wonder, can one even be sure a lifetime value calculation is accurate enough to be useful? That's where AI comes in.
AI tools can help you characterize how different types of patients move through a health system, as well as develop more accurate estimates of lifetime value. These models can then be used by care providers in real time to inform patient outreach and disease management.
The challenges with lifetime value calculations faced in healthcare are similar to the challenges in the area of retail. Our e-commerce retail clients use predictive models that take into account demographics and site activity to provide a lifetime value estimate and assess churn risk. While e-commerce companies and healthcare providers operate differently, some of the same core data points and AI applications exist in both.
Win #3: Assessing The Likelihood Of A Desirable Clinical Outcome
Calculating the odds of a healthy outcome for a specific patient requires aggregating data on patient demographics and engagement, as well as key clinical data. If this data is already being collected, implementing an AI solution will require structuring an algorithm based on these key data points.
Manifold worked with a chronic disease management company to implement a similar process. We developed predictive models that assessed the likelihood of patients meeting desired clinical outcomes, and the models assigned each patient with a “risk score.” By integrating the risk score into the staff's workflow and a consumer-facing mobile app, our client was able to develop more personalized treatment plans. The result was great operational efficiency, a better patient experience, and improved outcomes overall.
AI garners a lot of attention in the press, and that can tempt healthcare leaders to dive in and initiate a rapid, across-the-board implementation of AI technology. In the long run, however, starting small will provide the traction needed to create lasting solutions. Accessible, incremental wins add to an organization’s momentum, and when there are several in a row, your key stakeholders will start to notice.
About The Authors
Vinay Seth Mohta is a managing director at Manifold, an artificial intelligence product development studio with offices in Boston and Silicon Valley. Prior to his work at Manifold, Vinay was the CTO, CISO, and co-founder at Kyruus, which uses data to help health systems match patients with providers more precisely. He has also served as a product manager for travel brand Kayak and as CTO for the Global Health Delivery Project, a nonprofit collaboration that supports healthcare professionals dedicated to value-based care. Vinay earned a Bachelor of Science in computer science and a Master of Engineering in electrical engineering, both from the Massachusetts Institute of Technology.
Sailu Challapalli is an advisor at Manifold who has more than 15 years of experience in healthcare strategy, technology, and operations. She is currently the senior director, real world evidence (RWE) at Flatiron Health, where she oversees product strategy and operations in leveraging RWE to answer critical clinical and research questions. Prior to joining Flatiron, Sailu was the vice president of account management at Kyruus, an enterprise software company that worked with health systems to improve patient access and referral management. She has also worked at McKinsey & Company and Columbia University Medical Center. Sailu received an MBA from Harvard Business School and her bachelor's and master's degrees in computer science and electrical engineering from the Massachusetts Institute of Technology.