A New Frontier In Remote Patient Monitoring (RPM): Gesture Detection
By Uri Schatzberg, Somatix
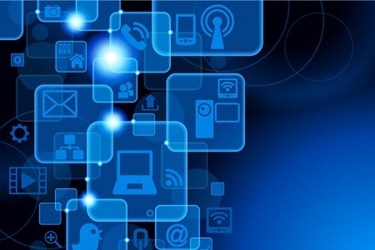
The healthcare market has been flooded with new RPM solutions. There are wired connections and wireless sensors tracking patients’ physical states, monitoring and alerting on patient deterioration, notifying caregivers if discharged or disabled patients or even elderly patients’ wellness indicators have changed for the worse — the need for remote monitoring is real. But we have only scratched the surface.
Many current remote monitoring solutions aim to enhance patient safety and prevent negative health outcomes. Such solutions often require proactive reporting of events by individuals or are limited to a predefined location. Additionally, in most cases, such devices are only able to detect — beyond simple fitness tracking — a single indicator like heartbeat, blood pressure or glucose measurement.
Healthcare innovation is in search of a passive, intuitive monitoring tool with a broader tracking scope. The answer to this search may be found in a totally untapped source of data — our hands.
People constantly use their hands, and there is a wealth of health data and information to be found in these daily gestures. This data can be collected and reflect multiple physical and emotional states. The sensors (mainly accelerometer and gyroscope) in today’s IoT-based devices like smartwatches or smartbands make it possible to detect a range of activities of daily living (ADLs): Have patients taken their medication? Had enough to drink? Eaten on time? Has a patient experienced a sudden fall? How is a patient’s smoking cessation program going? Have they lessened their smoking habit?
The proliferation of low power and low-cost sensors is enabling new and innovative applications in wearables and Internet of Things (IoT). Today, we see the emergence of IoT-connected wearables, mainly smartwatches and smartbands, equipped with sensors designed to recognize physical hand movements. These sensors recognize movement as an input which can then be translated into meaningful type data. Gesture has been accepted as a non-verbal form of communication that can be digitizing into multiparametric data. Yet, in order to fully capture and translate gesture, it is necessary to identify complex, three-dimensional movements, some of which are direct hand movements (i.e. drinking, eating or smoking) or indirect movements, including body motions characterized by unique hand gestures –meaning, how a person’s hand moves when they walk, run or fall down.
Wearables: What’s Inside?
All of this is made possible using a combination of wearables’ built-in sensors. These include Inertial Sensors, such as accelerometers and gyroscopes. Accelerometers are electromechanical devices that measure acceleration forces. These forces may be static, like the constant force of gravity pulling at your feet, or dynamic, caused by moving or vibrating the accelerometer. Gyroscopes are used for measuring the rotation speed, or the degrees per second the hand has turned. Together these two sensors can recognize a hand gesture in physical space.
To be able to translate the data collected by the inertial sensors and gain a full understanding of a person’s health indicators, some supplemental information may be needed, derived from additional sensor types. For example, ambient sensors inform us on environmental parameters including weather, and bio sensors provide personalized biological indicators such as heart beat or blood pressure.
Data With A Purpose For Timely Intervention And Improved Care
Simple data collection is not enough. To be able to provide healthcare professionals with meaningful insights about their patients, a detailed analysis must be performed. This requires the application of adaptive Machine Learning algorithms as well as advanced Big Data analysis to accurately identify significant behavior patterns, such as times when elderly or discharged patients need to take their medication or how many glasses of water they are required to drink on a hot summer day. By understanding these behavior patterns, we can also recognize, for example, a patient’s depression, which can be related to too much eating or no eating at all, too much sleep or no sleep at all.
Gesture detection has the ability to illuminate a wide array of health circumstances and conditions. For example, devices with geo-fencing capabilities can identify elderly patients suffering from dementia leaving their designated “safe” locations. Or, by identifying the specific hand-to-mouth gestures of adolescents with eating disorders, caregivers can track overeating or patients who are not meeting their daily caloric requirements.
Recognition of movements and gestures is bound to play an important role in remote patient monitoring solutions. The ability to recognize hand gestures will allow caregivers to use this analyzed information to determine patients’ overall wellbeing. This novel, yet highly intuitive approach will become a critical tool for monitoring patients across the continuum of care and providing intervention at patients’ point of need. A combination of advanced sensor technology, algorithmic intelligence and human behavioral habits can become the key to delivering better, personalized, real-time care at a significantly reduced cost. Achieving this will be a major milestone on the mission to empower positive healthcare transformation.
About The Author
Uri Schatzberg, CTO & Co-founder of Somatix, is a passionate technology leader. In recent years his focus has been on the development of Somatix’s gesture detection algorithm based on data collected from wearable devices (IoT). He gained his vast experience working for companies like Intel Corporation, Horizon Semiconductors and more, where he has been involved in developing digital communication algorithms and modems (2G, 3G, DOCSIS 3.0), and later specialized in Wi-Fi and inertial indoor navigation, data fusion and non-linear filtering. Uri has a MSc, BSc and MBA from Tel Aviv university. He has authored four publications and 20 patents.