A Better Data Governance Model
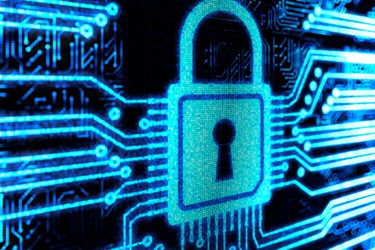
Three key strategies for advancing analytics and health information exchange
By Barbara Antuna, MD, FACEP, ABPM-CI, Medical Informatics Specialist, Wolters Kluwer Health Language Solutions
Access to timely, accurate information is foundational to smart decision-making. While most industries have long recognized the value of data-driven operations, healthcare is later to the game when it comes to the concepts of Big Data and analytics.
Current industry movements around the Triple Aim of improved population health, patient experience, and costs are driving uptake of analytics strategies to leverage the power of data. Yet, even as the healthcare industry at large rallies behind the promise of population health management and health information exchange, one critical part of an effective strategy often falls short in today’s health systems — data governance.
Analytics strategies are meaningless if the data powering them is unreliable. Hospitals and health systems may be sitting on terabytes of data, but many lack the governance models needed to extract quality information that drives more strategic decision-making.
Across the board, IT professionals need to take a closer look at how data is managed at their organizations. Without a governance model that addresses standardization and quality management of data elements, healthcare organizations are limited in their ability to reach the level of interoperability and achieve the high-level information exchange and analytics required to succeed in value-based care.
Thus, forward-looking healthcare organizations that are currently moving full speed ahead on the analytics train are wise to pull the brakes, take a breath, and assess whether they have a meaningful data governance strategy in place. This begins with top-down prioritization and a strategy that speaks to data creation, storage, normalization, analysis and reporting. And while healthcare organizations can consider a variety of tactics, all effective governance models address the following three areas.
- Ownership
A common pitfall to effective data governance in today’s healthcare organizations is lack of ownership. This function is often a subject of debate between IT and the various departments or business entities using the data — clinical specialties, financial functions, providers, and vendors to name a few.
As a best practice consideration, ownership should fall to the primary users of specific data groups since they have a deep and strategic understanding of those elements. While data is managed within an IT infrastructure, IT professionals are best leveraged as partners in the ownership equation. Otherwise, many organizations end up with siloed departmental data that resides in disparate systems and lacks organizational-wide standardization. The end result is that the same data element may be represented in numerous ways across systems, creating challenges to accurately aggregating data for analytics initiatives and quality reporting.
Alternatively, when IT professionals shepherd data owners across various departments and business units to define standards for all internal and external data, healthcare organizations achieve consistency in data representations, creating a single source of truth. Ultimately, this framework minimizes negative downstream impact, delivering better return on quality measures or other data elements that may impact reimbursement.
- Understanding All Organizational Data Elements
Before data can be effective governed, it must be identified. Thus, effective strategies start with IT and data owners working together to inventory of all data elements including standardized reference classifications and local system terminologies. Once identified, data elements are maintained in a catalogue, enabling organizations to efficiently target management and maintenance efforts.
Healthcare organizations run the risk of missing key data when this critical step is overlooked. A thorough analysis entails an inventory of all software applications and data files and end with the establishment of written policies detailing how, when, how often and by whom it should be updated.
- Implement An Ongoing Change Management Process
In healthcare, there is one constant — change. As the industry advances, so do industry standards and the classification systems that power clinical terminology.
To ensure data is current, accurate and complete for analytics and health information exchange, data governance must entail formal and ongoing processes for updating data as needed. Policies and procedures may address changes to such areas as data models, definitions, or structures as well as stewardship responsibilities. In addition, healthcare organizations must understand what is changing and how it impacts operations. For example, a change to a new condition code may impact downstream analytics for population health.
In reality, few organizations outside of large, academic facilities and established health systems have mature data governance strategies that truly align with analytics needs of today’s healthcare climates. Competing initiatives often create resource barriers to prioritizing these initiatives, yet, data governance provides the foundation needed to successfully move industry initiatives forward.
In light of these challenges, hospitals and health systems are increasingly looking to automation and advanced terminology management infrastructures to provide the backbone to data governance workflows. Solutions and tools exist that provide the front-end data capture and normalization capabilities needed to standardize data elements around a single source of truth.
By eliminating time-consuming manual processes associated with managing information across a multitude of disparate systems, this strategy of technology and workflow governance positions resource-strapped IT departments for greater success with meaningful data governance. The most advanced solutions provide the software, content, and consulting expertise needed to effectively map, translate, update, and manage standard and enhanced clinical terminologies on an enterprise scale.
The need for meaningful data governance is greater than ever, and the stakes are high for implementing high-level analytics and frameworks to support health information exchange in the value-based climate. Healthcare organizations must prioritize these strategies as a critical first step as they align with current industry movements.