Predictive Analytics And AI Trends Impacting Healthcare IT In 2021
By Jorge Torres, MindsDB
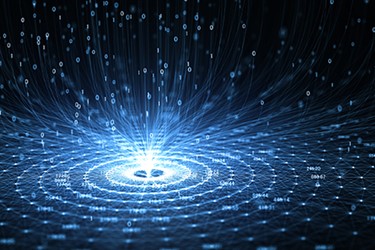
Artificial intelligence and predictive analytics continue to make transformational changes throughout the business world. While healthcare IT is seeing some impacts from AI and machine learning, it lags behind other industry sectors in fully adopting this emerging technology. However, necessity is sometimes the mother of invention, as evidenced by the Coronavirus pandemic still ravaging the planet.
Because of the strain and unpredictability COVID-19 is causing hospitals and medical establishments, faster adoption of AI is now critical. Unfortunately, healthcare IT departments suffer from a skills gap as well as other issues when implementing new systems using machine learning. Nonetheless, the demand for AI remains strong.
Here are some of the major 2021 trends related to AI in the healthcare industry. Anyone working in healthcare IT and related insurance carriers needs to take heed of this coming transformation.
AI Making Inroads In Healthcare Over The Last Year
While not fully embracing the technology, the healthcare industry has seen some impacts from AI over the last year or two. For example, at-risk populations benefit from the AI-powered monitoring of chronic issues. This area especially holds promise for the treatment of COVID-19 patients; illustrating how machine learning models have the potential to augment the decision-making processes of medical professionals. Additionally, this proactive monitoring approach prevents costly emergency hospital trips.
To better gauge the current use of AI and machine learning within the healthcare insurance field, our team surveyed technology professionals working in that arena. In this case, 50% of the respondents noted a current involvement with projects using either predictive analytics or machine learning. Another point of interest is less than 6% feel they boast advanced knowledge of ML.
As noted earlier, COVID-19 is driving the increased adoption of AI and ML in healthcare. One benefit is improved decision-making regarding patients, both in diagnosing the virus and in treatment. Additionally, predictive analytics helps healthcare organizations improve operational efficiency to better handle a physician shortage exacerbated by the pandemic.
AI also helps doctors better diagnose diseases by using image analysis powered by machine learning algorithms. It’s another example of how ML enhances the ability of the medical professional. This image interpretation use-case applies to X-Rays, angiograms, CT, and MRI scans.
Improving Efficiency In Hospital Operations
In addition to helping hospitals streamline their operations, AI also provides notable benefits in the area of revenue cycle management. Dr. Sanjay Basu, Collective Health’s Director of Research and Analytics, feels this boost in efficiency allows them to concentrate on providing better patient care.
“AI reduces the task of manually reviewing thousands of medical claims, and instead focuses our staff on performing warm, human outreach, and thinking through complex problems together with our members,” said Basu.
Additionally, machine learning algorithms help hospitals perform information retrieval more effectively. These ML-powered software search agents automatically ensure patient or other data is up to date. As the use of EMR for patient data becomes more commonplace, expect AI to play a significant role in ensuring efficient data management.
Predictive Analytics Provides Benefits In Healthcare Insurance
Artificial intelligence and machine learning currently provide significant advantages to companies in the financial and insurance sectors. So, it stands to reason that health insurance companies would also benefit from using AI and ML. Automated ML-powered systems for managing patient policy data boost overall efficiency. Increased adoption of AI-driven technology within the healthcare insurance sector is an important trend for 2021.
At a high level, three major applications for machine learning in health insurance are predicting high-cost claims, identifying at-risk policyholders, and better efficiency in managing both patient care and costs. Not surprisingly, these benefits largely parallel those now occurring throughout the insurance industry as a whole. In fact, a report by Accenture predicted insurance providers will save up to $7 billion in administrative costs over 18 months due to leveraging AI.
A smarter and more efficient claims process becomes possible when adopting AI technologies. A recent study by McKinsey notes that insurance companies flag 80% of all submitted claims as potentially fraudulent. Using trained machine learning models on masses of data helps detect patterns used to improve claims fraud detection efforts. As such, expect more insurers to take advantage of ML for battling fraud in 2021 and beyond.
As the survey noted earlier, slightly over half of all respondents in the health insurance industry are currently working on projects in either predictive analytics or machine learning. Nearly 80% of the professionals building these projects are data scientists or data analysts. Typically, the project data resides in either a data warehouse or a relational database system. Notably, the most widely used databases are Oracle, MySQL, and MongoDB.
What Trends Are Hampering The Adoption Of AI And ML In Health Insurance?
With all the obvious benefits of AI and machine learning in healthcare IT, why is the industry lagging behind other business sectors in adopting this emerging technology? The reasons for this lag are numerous. An obvious one involves a skills gap in IT professionals when it comes to AI and ML. Additionally, they don’t have enough practical experience to wean meaningful insights from the data.
According to the survey of healthcare insurance technology professionals, nearly half only have beginner-level machine learning knowledge. As we noted earlier, less than 6% boast expert-level ML skills. In this situation, focused training in AI and machine learning is an obvious help. Using an advanced tool that makes high-level machine learning routines accessible to professionals who aren’t data scientists or programmers offers another viable solution.
Difficulty in accessing data also hampers efforts for health insurers to reap the benefits of AI technologies. Once again, the survey highlights this issue. As highlighted earlier, most health insurance data resides in either a data warehouse or a relational database. These systems generally require specialized knowledge to perform queries, let alone run a machine learning model against the data.
In around half of the current healthcare insurance providers, data scientists are the ones producing results from predictive analytics and machine learning projects. These are highly-trained professionals, with specialized experience and, in most cases, an advanced degree. Putting the power of AI in the hands of more people within the organization leads to wider adoption of these emerging technologies. This is especially valuable with machine learning empowering domain experts in the areas of healthcare and insurance.
Ultimately, any data-driven hospital or medical office needs a centralized data strategy. It’s an approach that benefits patients by improving diagnoses and the overall standard of care. Additionally, a boost in operational efficiency saves costs for both medical organizations and health insurance providers. This strategy helps companies working in healthcare truly reap the benefits of AI and machine learning.
The Benefits Of An Open-Source AI-Layer For Databases
Remember, data for many healthcare organizations remains difficult to access; requiring the skills of a data scientist to wean actionable information. In this scenario, an easy-to-use AI-layer able to connect to and query a database offers significant benefits. Users execute ML models with a simple SQL query. They receive relevant results along with an explanation of how the model derived its result set.
Making the power of AI/ML-driven solutions easily accessible to medical and insurance professionals improves the adoption of these nascent technologies. The ultimate result is increased efficiencies and improved benefits for the healthcare industry.
About The Author
Jorge Torres (Co-founder and CEO of MindsDB) is an entrepreneur, academic, AI/machine-learning expert, and Visiting Research Scholar at the University of California, Berkeley. Before Co-Founding MindsDB Jorge was a senior software engineer at CareJourney and Skillshare and the second full-time developer at CouchSurfing.com - a platform he helped to grow from a few thousand to a few million users. Jorge specializes in creating software that transforms cultural exchange and is passionate about democratizing Machine Learning. His new venture MindsDB completed the YCombinator Accelerator program in March 2020 and recently announced their $4 million seed funding round led by OpenOceanVC.