Personalized PTSD Diagnosis Tool Helps Predict Risk

By Christine Kern, contributing writer
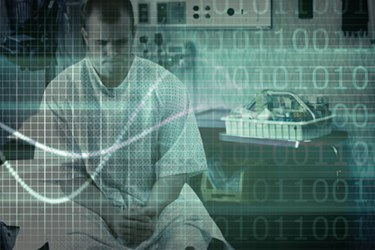
NYU researchers have created a personalized PTSD diagnosis tool.
Researchers from New York University’s Langone Medical Center and the Danish Veteran Center in Ringsted, Denmark have created a personalized PTSD diagnosis tool to identify 800 different ways people are at increased risk for being diagnosed with the disorder. Results from the study were published by BMC Psychiatry.
“Our study shows that high-risk individuals who have experienced a traumatic event can be identified less than two weeks after they are first seen in the emergency department,” study co-author Arieh Y. Shalev, M.D., professor of psychiatry at NYU Langone said. “Until now, we have not had a tool – in this case a computational algorithm – that can weigh the many different ways in which trauma occurs to individuals and provides a personalized risk estimate.”
Current computation methods to help clinicians diagnose PTSD are capable of calculating the average risk for entire groups of survivors, not granular enough to serve as individual risk prediction tools. The new algorithm applied risk prediction tools, currently used to predict the growth of cancer, to predict PTSD. Researchers found that, when applied to data collected within 10 days of a traumatic event, the algorithm can more accurately predict who is likely to develop PTSD.
“Until recently, we mainly used early symptoms to predict PTSD, and it had its drawbacks,” said Shalev. “This study extends our ability to predict effectively. For example, it shows that features like the occurrence of head trauma, duration of stay in the emergency department, or survivors’ expressing a need for help, can be integrated into a predictive tool and improve the prediction.”
Shalev added that devising a strong predictive model also is imperative for tailoring prevention efforts for people at risk for developing PTSD. He also said this latest publication builds upon data originally collected for an earlier study, and it is a “proof of concept” paper. For robust prediction across conditions, he says, the identified algorithm needs to be used to gather knowledge gained in traumatic events experienced by other patient populations and traumatic events.
The research team is already in the process of building a generalized predictive model, utilizing datasets from 19 other centers worldwide in a National Institute of Mental Health-funded study designed to produce a comprehensive predictive algorithm. “In the future, we hope that we will be better able to tailor treatment approaches based on more personalized risk assessment,” Shalev says. “PTSD exacts a heavy toll on affected individuals and society.”