Data Analytics: Making Healthcare Healthy, Wealthy And Wise
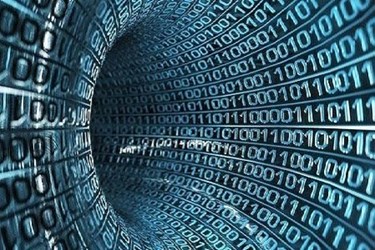
By Chetan Manjarekar, SVP and Head of Enterprise Solutions Group (ESG), Syntel, Inc.
An individual’s healthcare data is unchanging and will always be relevant. Information about a patient who underwent surgery at age 10 will still be relevant when he or she applies for insurance as an adult. Unique, complex, structured, unstructured, collected from many sources, and available in a variety of forms — healthcare data is a maze of information that must be filtered, deciphered, and analyzed.
Today’s technology not only allows the storage of massive amounts of data, but also enables fast analysis and real-time pattern detection that improves clinical efficiency, care quality, affordability and policy making.
Analytics are also central to achieving the systematic quality improvements and cost reductions demanded by healthcare reform, but this new age of analytics requires a foundational set of analytical information systems that many executives have not anticipated.
How will the healthcare industry leverage Big Data solutions? How can analytics be applied to data security challenges? Some industry players have successfully introduced a Healthcare Analytics and Research Framework to address some use cases that help improve outcomes and optimize costs.
Wellness Analytics
At every stage of the care continuum, there are many wellness or quality of life initiatives that may help either prevent or slow the progression of a health condition or disease. With a Healthcare Analytics and Research Framework, disease-specific profiling can be used to determine the course of care.
In cases of diabetes, the framework takes in a de-identified EHR dataset that includes details about diagnosis, allergies, lab results, medications, immunizations, vital signs and health behavior of members. Using advanced classification methods like Decision Trees, Support Vector Machine (SVM) and other analytical models, the framework can generate a probabilistic score for each member, indicating the likelihood of the person developing Type 2 Diabetes.
“At-risk” members can be identified and wellness plans can be created ahead of time, encouraging members to engage in healthy activities that reduce their risks.
Risk Management
Patients with chronic diseases account for a majority of U.S. health spending, despite a significant portion of morbidity and mortality being preventable. One of the key aspects of risk management in healthcare is identifying the likelihood of hospital admissions and estimating the predicted length of stay (or “LoS”). High-risk patients typically require longer LoS and more expensive medical procedures, driving up the cost of care.
Analytics platforms can sift through clinical data to help segment patients with chronic conditions into target groups that need dedicated focus and regular interventions. At the time of discharge, accurately identifying these high-risk/high-cost patients can help plan better follow-ups and more effective care management which will generate better patient outcomes and reduce costs.
Identifying Early Onset And Timely Intervention
Another form of risk management is to minimize the need for procedures and reduce the potential damage to patients through timely interventions.
For example, diabetes is a progressive disease, and one of its most pernicious effects is diabetic retinopathy, a leading cause of blindness. However, care effectiveness improves dramatically with early identification and intervention.
A well-designed analytics platform has the ability to analyze retinal image data and assign numeric values to the height, width and density of black spots, blood vessel thickness and mean and ratio of eyes to normalize the picture. This numeric dataset can be sent to the analytics framework to classify patients into diagnostic categories from zero (no retinopathy) to four (maximum retinopathy), quickly identifying those most in need of treatment.
Inpatient Classification And Prediction
An effective healthcare analytics framework should include algorithms that can chew through mountains of historical claims data to predict whether individuals are likely to be hospitalized within the next one to two years, including their estimated LoS. These solutions can also predict risk as a factor of socioeconomic status and patient behavior.
From a Payer perspective, identifying high risk patients helps develop and refine value-based health plans and enables preventive measures to minimize admissions and reduce the number of ER visits.
Readmission Analytics
A large percentage of readmissions are preventable if the discharge or transition has been planned carefully. Readmissions not only lead to penalties for healthcare providers, but also lead to significant costs in terms of subsequent admissions and treatment costs.
A well-constructed analytics framework should include 30 day re-admission algorithms that take inputs such as claim details, secondary illnesses, Charlson Comorbidity Index, diagnosis, discharge disposition, case notes, lab results, medications and vital signs.
Using advanced machine learning on supervised or unsupervised classification and prediction such as multilayer perceptrons, Naive Bayes classifiers, instance-based learning, Support Vector Machine and Logistics Regression models, the analytics framework can generate a probabilistic readmission score for each member. The high-risk categories are provided immediate attention.
Tread Carefully
Although there are many potential advantages to leveraging Big Data analytics in healthcare, the practice is not without its own set of challenges.
In January 2015, President Obama highlighted both the potential and the pitfalls of Big Data in healthcare, calling it “one of the greatest opportunities for new medical breakthroughs that we have ever seen.”
In unveiling his Precision Medicine Initiative, he called for the creation of a multi-stakeholder group including patients, bioethicists, privacy and civil liberties advocates, and technologists to identify and address any legal and technical issues related to privacy and data security in medicine.
In the same way, today’s health industry players must strike a balance between harnessing the power and potential of Big Data, while respecting patient privacy in a world where data collection will be increasingly ubiquitous, multidimensional, and permanent.